Virtual critters evolve bodies that help them learn
These creatures may inspire new ways to build robots
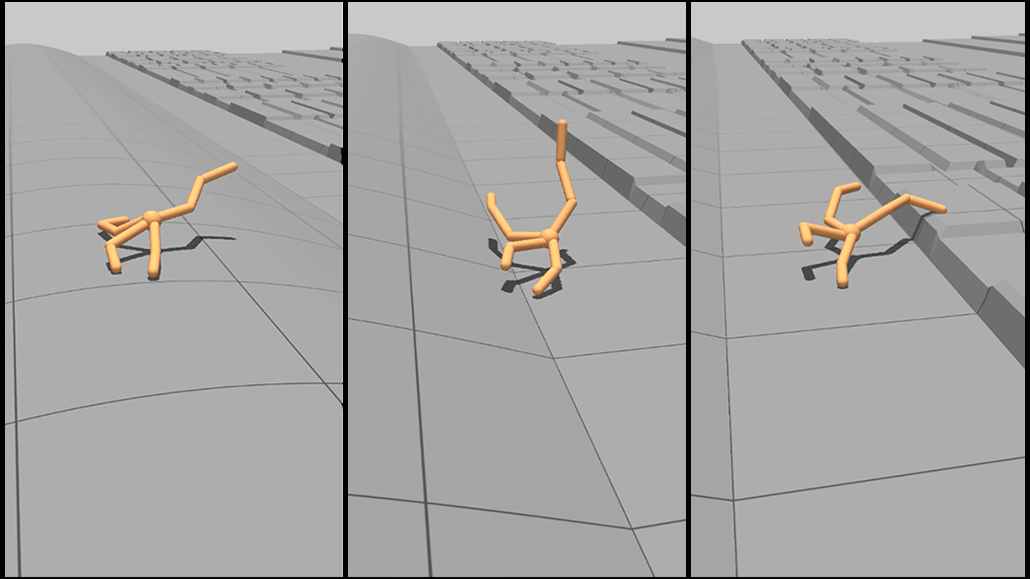
This virtual animal evolved long, paired limbs to help it move across a bumpy landscape as quickly as possible.
A. Gupta
A virtual creature swings four tentacle-like arms, pushing itself forward. It creeps up a hill then rushes down the other side. It looks like “an octopus walking on land,” says Agrim Gupta. This strange critter evolved its own body. It also learned its own method of moving. This mix of evolution and learning could help engineers build new kinds of robots, Gupta says.
A PhD student studying computer vision at Stanford University in California, Gupta is sort of like a grandfather to this octopus-like creature and hundreds of other odd-looking virtual critters. He created the ancestors that gave rise to these creatures. He calls them unimals, which stands for “universal animals.” That term reflects the fact that they can evolve into so many different body shapes. Some resemble real animals. Others are quite bizarre.
The team discovered that an unimal’s body type affects its ability to learn new things. We tend to think of learning as something that happens in the brain. But, Gupta notes, “your body plays a huge role in what things you can learn.” The type of world you live in matters, too.
If robots could evolve in a simulation, they might develop their own forms that work even better, Gupta and his colleagues thought. Then engineers could build bodies they never would have dreamed up on their own.
So they tried it out. Unimals that learned to move in more complicated simulated worlds ended up with bodies better suited for learning. Gupta and his group described this in Nature Communications last October.
“I was excited about this work,” says Sam Kriegman. He was not involved in this research but knows a lot about the topic. He works on evolutionary robotics at the Wyss Institute. It’s part of Harvard University in Boston, Mass. He also works at the Allen Discovery Center of Tufts University in Medford, Mass. Robot engineers have tended to copy bodies they see in nature. That’s why many robots resemble real animals, such as dogs or people.
Flailing around
An animal species evolves with small, random changes to its genes. Those changes that give it new advantages make it easier to survive. Computer scientists can now mimic this process in code. Here’s how Gupta’s team did it.
To start out, they gave their unimals bodies that look a lot like animal stick figures. Each has a single round head. Straight segments stick out of this head. They branch off into other segments, forming body parts that resemble arms, legs or tentacles.
Just over 500 randomly generated unimals get tossed into a virtual world, which is a lot like a video game. In the simplest game, each unimal has to cross a flat landscape. It figures out how to move using a computer model of machine learning. Machine learning is a type of artificial intelligence (AI) that allows computers to practice a skill until they have mastered it.
In this case, the machine-learning model controls the unimal’s body. At first, when the model knows nothing about moving, the body flails around as it tries out random motions. If one motion brings the unimal closer to its goal of crossing the landscape, the model learns to repeat that motion. The farther the unimal gets across the landscape, the higher its score in the game.
A bouncing starfish
Later, the unimals get split up into groups of four. Whichever member of the group has the highest score gets to evolve. Let’s imagine that the winner looks a bit like a starfish. When it evolves, its body changes in a random way. For example, it might lose some of its legs. Or, all of its legs might grow a new segment. Or one might get longer and another shorter. In this last case, the limbs get lighter. Then “the starfish can bounce around more easily,” Gupta explains.
Later, all unimals from the original group of four go back into the flat virtual world together with the new starfish. They remember nothing from their first trip through the world. They all have to start from scratch, flailing around until something works. Again, they all get a score and face off in groups of four to see who gets to evolve next.
This process repeats, over and over. Whenever a new unimal gets created, the oldest one dies. If it was doing a good job, then it will have evolved a few times before it died. That means it left behind a bunch of children and grandchildren that might do even better. Over many generations, unimals get better and better at crossing the landscape. They remember nothing from past experiences. That’s because the point isn’t to cross the landscape. It’s to evolve bodies that are better at learning to move.
Facing challenges
The flat world was just the beginning. Gupta and the team went through the same process again with new groups of random unimals in a bumpy landscape. And in a third world, the unimals had to push a cube to some target across a bumpy landscape. This was especially hard to master. By combining learning and evolution, however, unimals emerged that could handle it. One evolved two hand-like limbs that it used to push the cube.
The team then put all the unimals to the test in new types of worlds. These had obstacles that none had encountered before. They had to move up and down steep slopes. They had to push a ball to a target (which is a lot trickier than a cube since it can easily roll away). Again, the unimals remembered nothing of what they’d learned. All they had were body shapes that had worked well in one of the original three worlds.
Unimals that had evolved in the third world — the one with the bumps and the cube — “learned new tasks better and also much faster,” notes Gupta. Why? Their bodies had adapted to help them solve different types of problems.
For example, the unimal with hands could use those to push a ball. Unimals from the flat world had no need for hands, so had a far tougher time getting the ball under control. Having the right body, Gupta showed, “can greatly simplify the problem of learning a task.”
Engineers can’t always imagine the best body type for a certain robot. By combining evolution and learning, designers can generate and test thousands of new options. “We should use computers to help us be more creative and come up with new kinds of robot bodies,” says Kriegman.
It won’t be easy to move simulated creatures into reality, he adds. The real world is much messier and complex than a simulation. A body that works well in a computer may not work as well in real life. However, Kriegman says, “these problems are solvable.”