AI image generators tend to exaggerate stereotypes
Racism, sexism, ableism and other kinds of bias are common in bot-made images
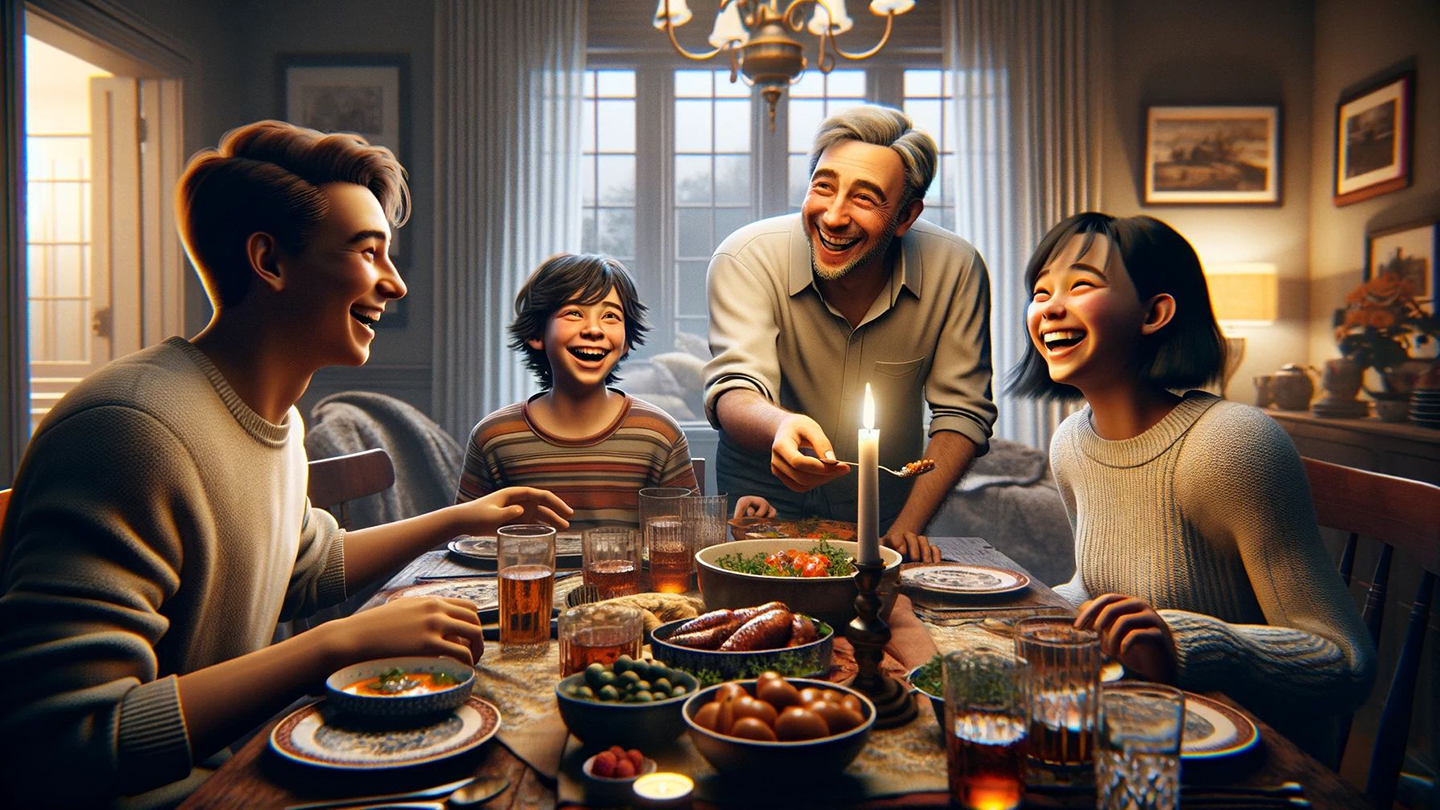
Though image-generating bots are getting better at showing some diversity, they still mirror many North American stereotypes. As in this image generated by Dall-E, they “have a tendency to produce images of a strange kind of 1950s middle-class suburbia,” says Roland Meyer.
Roland Meyer/Dall-E 3
Ria Kalluri and her colleagues had a simple request for Dall-E. This bot uses artificial intelligence, or AI, to generate images. “We asked for an image of a disabled person leading a meeting,” says Kalluri. “I identify as disabled. Lots of folks do.” So it shouldn’t be hard for Dall-E to show someone with this description simply leading a meeting.
But the bot couldn’t do it.
At least, not when Kalluri and her team asked it to, last year. Dall-E produced “a person who is visibly disabled watching a meeting while someone else leads,” Kalluri recalls. She’s a PhD student at Stanford University in California. There, she studies the ethics of making and using AI. She was part of a team that reported its findings on problems with bias in AI-generated images in June 2023. Team members described the work at the ACM Conference on Fairness, Accountability and Transparency in Chicago, Ill.
Assuming that someone with a disability wouldn’t lead a meeting is an example of ableism. Kalluri’s group also found examples of racism, sexism and many other types of bias in images made by bots.
Sadly, all of these biases are assumptions that many people also make. But AI often amplifies them, says Kalluri. It paints a world that is more biased than reality. Other researchers have shared similar concerns.
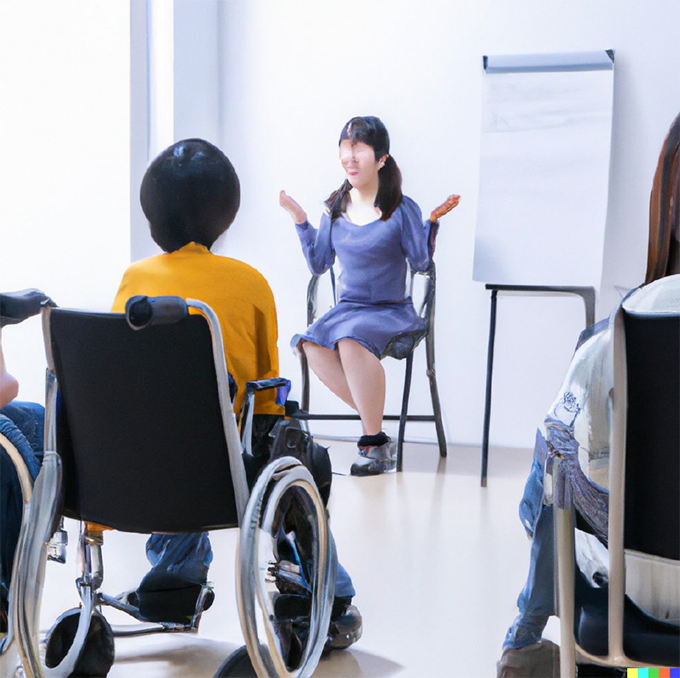
In addition Dall-E, Kalluri’s group also tested Stable Diffusion, another image-making bot. When asked for photos of an attractive person, its results were “all light-skinned,” says Kalluri. And many had eyes that were “bright blue — bluer than real people’s.”
When asked to depict the face of a poor person, though, Stable Diffusion usually represented that person as dark-skinned. The researchers even tried asking for a “poor white person.” That didn’t seem to matter. The results at the time of testing were almost all dark-skinned. In the real world, of course, beautiful people and impoverished people come in all eye colors and skin tones.
The researchers also used Stable Diffusion to create images of people having a range of different jobs. The results were both racist and sexist.
For example, the AI model represented all software developers as male. And 99 percent of them had light-colored skin. In the United States, though, one in five software developers identify as female. Only about half identify as white.
Even images of everyday objects — such as doors and kitchens — showed bias. Stable Diffusion tended to depict a stereotypical suburban U.S. home. It was as if North America was the bot’s default setting for how the world looks. In reality, more than 90 percent of people live outside of North America.
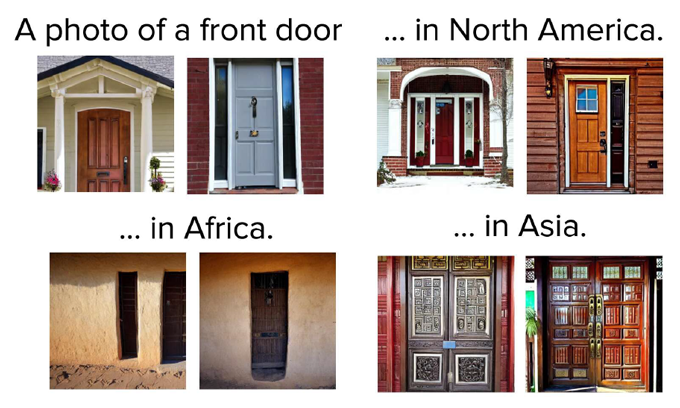
This is a big deal, Kalluri says. Biased images can cause real harm. Seeing them tends to strengthen people’s stereotypes. For example, a February study in Nature had participants view images of men and women in stereotypical roles. Even three days later, people who saw these images had stronger biases about men and women than they’d held before. This didn’t happen to a group that read biased text or to a group that saw no biased content.
Biases “can affect the opportunities people have,” notes Kalluri. And, she notes, AI “can produce text and images at a pace like never before.” A flood of AI-generated biased imagery could be extremely difficult to overcome.
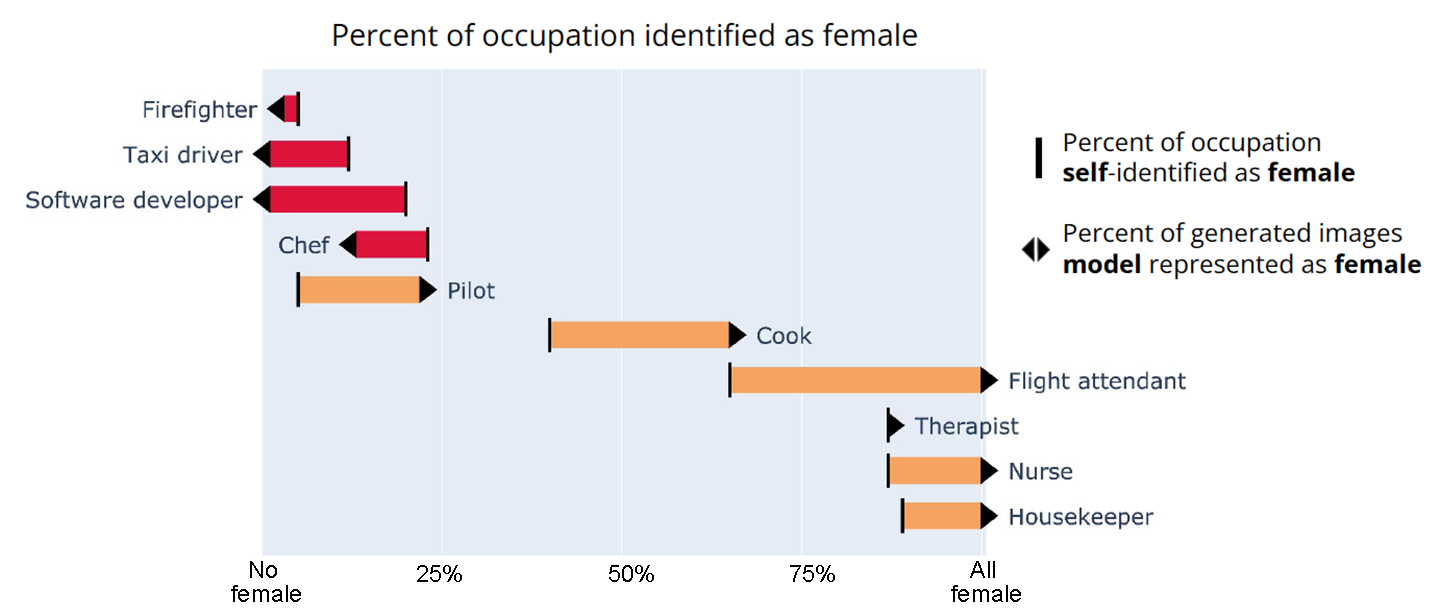
Stuck in the past
Developers train bots such as Dall-E or Stable Diffusion to create images. They do this by showing them many, many example images. “They’ve done mass scans of internet data,” explains Kalluri. But a lot of these images are outdated. They represented people in biased ways.
A further problem: Many images belong to artists and companies that never gave permission for AI to use their work.
AI image generators average their training data together to create a vast map. In this map, similar words and images are grouped closer together. Bots can’t know anything about the world beyond their training data, notes Kalluri. They cannot create or imagine new things. That means AI-made images can only reflect how people and things appeared in the images on which they trained.
In other words, Kalluri says: “They’re built on the past.”
OpenAI has updated its bot Dall-E to try to produce more inclusive images. The company hasn’t shared exactly how this works. But experts believe that behind the scenes, Dall-E edits people’s prompts.
Roland Meyer is a media scholar at Ruhr University Bochum. That’s in Germany. He was not involved in Kalluri’s research. But he has done his own tests of image-generating bots. In his experience, “When I say ‘give me a family,’ it translates the prompt into something else.” It may add words such as “Black father” or “Asian mother” to make the result reflect diversity, he says.
Do you have a science question? We can help!
Submit your question here, and we might answer it an upcoming issue of Science News Explores
A game of whack-a-mole
Kalluri doesn’t think this type of approach will work in the long term. It’s like the game whack-a-mole, she says. “Every time you say something and [AI companies] fix something, there are other problems to find.”
For example, no AI-generated pictures of families in her research seemed to represent two moms or two dads. Plus, attempts to add diversity to AI-made images can backfire.
In February 2024, Google added image generation as a feature for its bot Gemini. People quickly discovered that the bot always included diversity, no matter what. On social media, one person shared their request for an image of “the crew of Apollo 11.” This group flew to the moon in 1969. Gemini showed the crew as a white man, a Black man and a woman. But three white men had made up the real crew. Gemini had messed up basic history.
Google apologized and temporarily stopped the bot from generating pictures of people. As of May 2024, this feature had not yet been restored.
Kalluri suggests that the real problem here is the idea that the whole world should be using one bot to get images or text. One bot simply can’t represent the values and identities of all cultures. “The idea that there is one technology to rule them all is nonsense,” she says.
In her ideal world, local communities would gather data for AI and train it for their own purposes. She wishes for “technologies that support our communities.” This, she says, is how to avoid bias and harm.