Two AI trailblazers win the 2024 Nobel Prize in physics
John Hopfield and Geoffrey Hinton used brain-like networks to jump-start machine learning
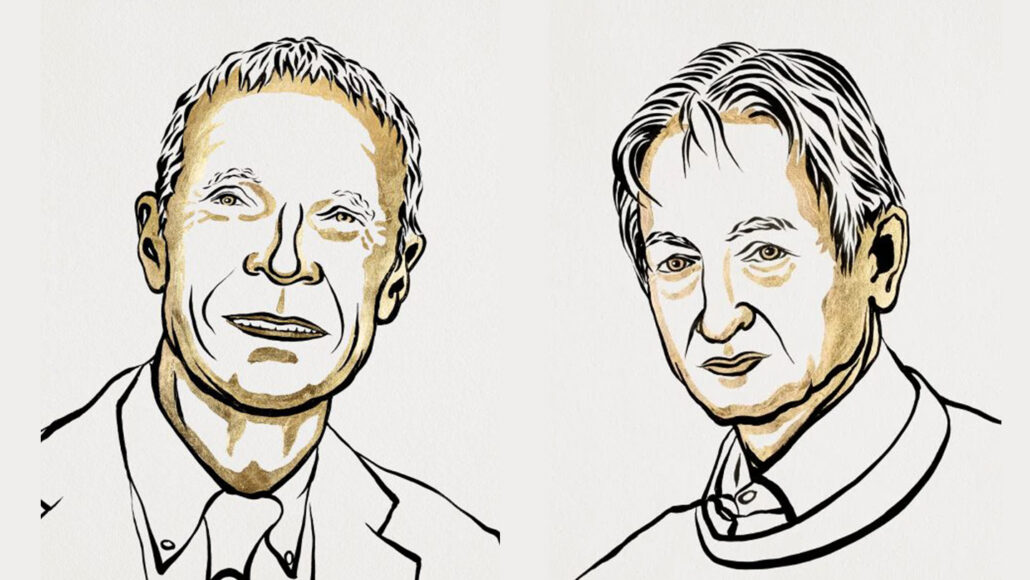
For discoveries that allow machine learning with artificial neural networks, John Hopfield (left) and Geoffrey Hinton (right) won the 2024 Nobel Prize in physics.
Niklas Elmehed, © Nobel Prize Outreach
By Emily Conover and Lisa Grossman
Artificial intelligence is showing up in nearly every aspect of our digital lives. On October 8, two of its trailblazers nabbed the 2024 Nobel Prize in physics.
They’re John Hopfield and Geoffrey Hinton. The two won “for foundational discoveries and inventions that enable machine learning with artificial neural networks,” the Royal Swedish Academy of Sciences in Stockholm says. These tools seek to mimic how the human brain works. Today, this tech underlies things like image-recognition systems, soccer-playing robots, large language models such as ChatGPT and more.
The prize surprised many. These AI developments typically are linked more to computer science than physics. But the Nobel committee noted that these neural-network tools were clearly based in physics.
“I was delighted to hear [of this award],” says AI researcher Max Welling. He works at the University of Amsterdam in the Netherlands. Indeed, he adds, “There is a very clear connection to physics.” These systems have been “deeply inspired by physics.”
What’s more, the discovery made many developments in physics possible. “Try to come up with a technology that has had a bigger impact on physics,” he says. “It’s hard.”
No one was more shocked by the new award than Hinton himself: “I’m flabbergasted. I had no idea this would happen,” he said by phone. That was during a news conference announcing the award.
“The work that Hopfield and Hinton did has just been transformative,” says Rebecca Willett. She’s a computer scientist at the University of Chicago, in Illinois. And it doesn’t just affect scientists developing AI, she adds. It’s transforming society.
Neural networks have helped scientists grapple with large amounts of complex data. This has given rise to many important achievements. They include making images of black holes and designing ingredients for better batteries. Machine learning also has made strides in biomedicine. It’s improving medical imaging. It’s also aiding an understanding of the role of proteins based on how they fold in the body.
The two winners will split the prize of 11 million Swedish kroner (equal to about $1 million).
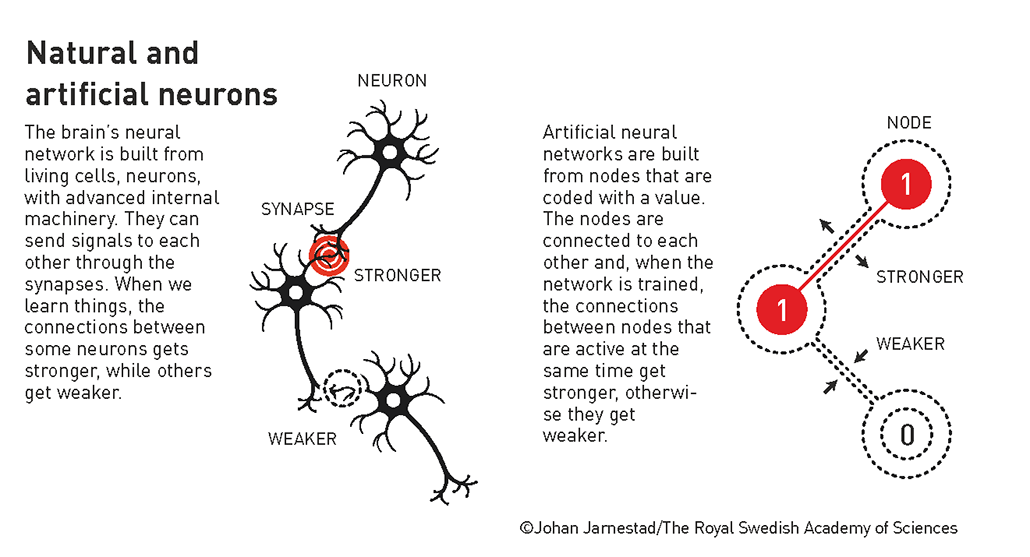
Networks become experts at finding patterns
Neural networks look for patterns in data. They’re based on a web of individual features called nodes. These nodes have been inspired by individual neurons in the brain. Feeding data to these nodes trains the network to find patterns. This process sets the strengths of the links between nodes. In this way, the network learns to draw accurate conclusions.
Hopfield works at Princeton University in New Jersey. In 1982, he created an early type of neural network. Called a Hopfield network, it could store and reconstruct patterns in data. This network relied on a concept something like the small magnetic fields in magnetic materials. Atoms in those materials have magnetic fields that can point up or down. Those are like the 0 or 1 values at each node in a Hopfield network.
For any given pattern of atoms in a material, scientists can determine its energy. A Hopfield network can be described by a similar “energy.” A Hopfield network minimizes that energy. In this way, it can find patterns hiding in those data that had been fed into it.
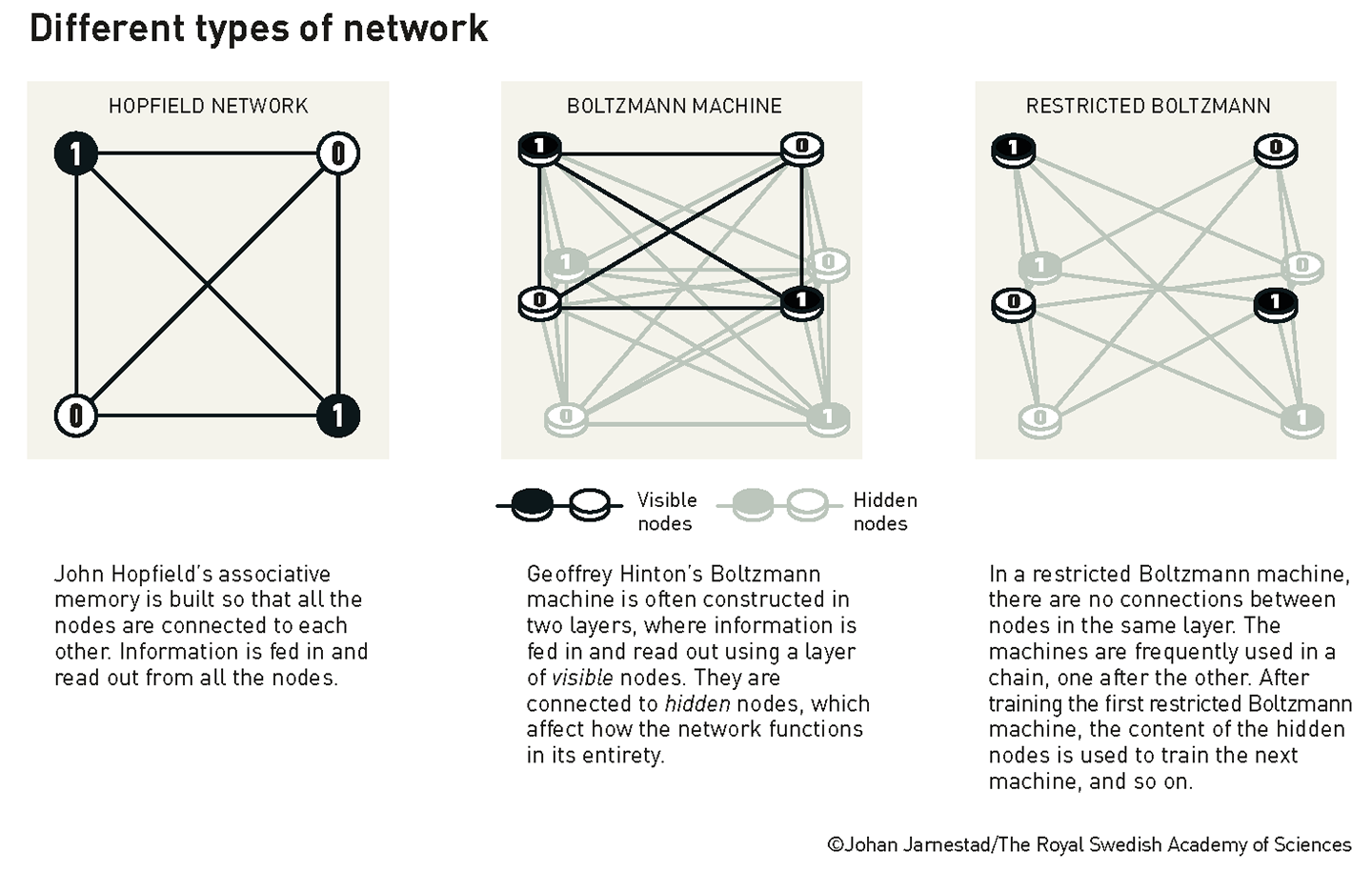
Hinton, who works at the University of Toronto in Canada, built on that technique. He developed a neural network called a Boltzmann machine. It’s based on statistical physics (including the work of 19th century physicist Ludwig Boltzmann). Boltzmann machines contain some hidden nodes. These are nodes that don’t directly receive inputs. They take in data from other nodes and help process it.
Different possible states of the network have different likelihoods of showing up. These probabilities are set by what’s known as a Boltzmann distribution. It describes properties of many particles (such as molecules in a volume of gas).
Since the 1980s, researchers have vastly improved upon these AI techniques. AI systems have also become far more complex. Deep-learning models now have many layers of hidden nodes. Some boast hundreds of billions of connections between nodes.
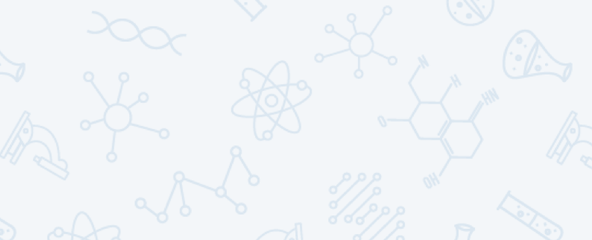
Educators and Parents, Sign Up for The Cheat Sheet
Weekly updates to help you use Science News Explores in the learning environment
Thank you for signing up!
There was a problem signing you up.
Costs to this AI as well as benefits
AI based on neural networks is capable of feats that could not be imagined in the 1980s. Yet this tech also poses risks. Many researchers are now investigating how the wide use of machine learning might harm society. Some have uncovered risks that it can reinforce racial biases. Ample evidence shows that it has made it easier to spread misinformation. In much the same way, AI can make plagiarism and cheating quicker and easier.
To better train AI systems, researchers have scraped the internet for text or images. That involves truly vast amounts of data. This training requires likewise vast amounts of energy and other resources.
Some scientists, Hinton included, also worry that AI could become too smart.
But other scientists challenge the idea that AI is on a path to world domination. Plenty of AI models have been making laughable mistakes that defy common sense.
In the near term, though, there is a real danger of AI putting people out of work and spreading misinformation. “I think those are very real concerns in the here and now,” Willett says. “That’s because humans can take these tools and use them for malicious purposes.”
Do you have a science question? We can help!
Submit your question here, and we might answer it an upcoming issue of Science News Explores