Explainer: What is statistics?
Most scientific research couldn’t glean meaning from data without the help of statistics
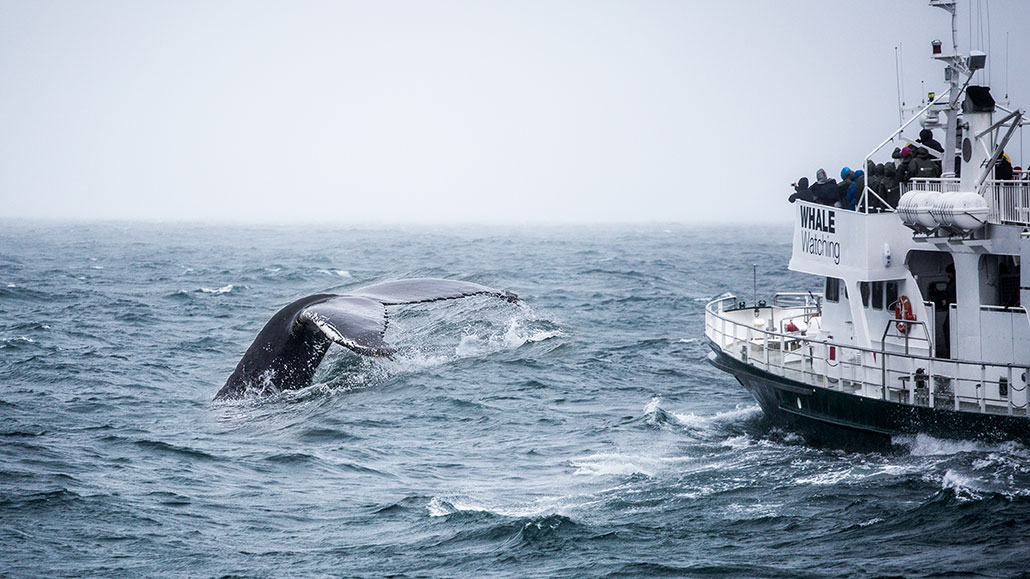
An isolated numerical fact can be a statistic. The field of statistics is different. It uses data sets to scout for patterns between things, looking to find a causal link, such as whether whales exposed to noise from boats (as here) have fewer babies.
Francesco Riccardo Iacomino/iStock/Getty Images Plus
When describing statements with numbers, people often refer to them as statistics. For instance, if 70 out of 100 students got a B on an English test, that would be a statistic. So would the make-believe statement “90 percent of toddlers love tuna.” But the field of statistics involves much more than a collection of factoids.
Statistics is a different kind of animal than other fields of STEM. Some people consider it to be a type of math. Others argue that while statistics is like math, it’s too different from math subjects to be viewed as part of that field.
Researchers see data all around them. Data are waiting to be gathered from penguin poop and the weather outside. They lurk in the motion of planets and talks with teens about why they vape. But these data alone don’t help researchers get far. Scientists need to think through how they structure their studies to glean meaningful information from these data.
Statistics helps them do that.
It has helped paleontologists figure out how to tell if a fossil belonged to a male or female dinosaur. Statistics has helped researchers show that medicines are safe and effective — including the COVID-19 vaccine.
Researchers in statistics are called statisticians. They hunt for patterns in data. Statisticians can use data collected from a few bottlenose dolphins to make interpretations for other dolphins of the same species. Or they can look for connections over time between carbon-dioxide emissions and fossil-fuel use. They can use those connections to estimate how future CO2 levels might change if use of fossil fuels rises, falls or remains about the same.
“I have skills marine biologists need — and those skills are statistics,” says Leslie New. She is a statistical ecologist at Washington State University in Vancouver. New uses statistics to study marine mammals, such as whales and dolphins.
She uses statistics to explore relationships between disturbances and marine-mammal populations. These could be things like ship sounds. They could also be problems that arise from nature — like more predators or less food.
One of the main statistical tools New uses is called state-space modeling. It “sounds fancy and the details of it can get very, very persnickety,” she notes. But there’s one basic idea behind it. “We have things that we’re interested in that we can’t see. But we can measure parts” of them, she explains. This helps researchers study an animal’s behavior when they can’t see the animal in question.
New shared an example about eagles. Scientists can’t follow a golden eagle on its migration from Alaska to Texas. That makes data about how often the bird stops to rest, forage and eat seem like a mystery. But researchers can attach trackers to the bird. Those devices will tell the researchers how fast the eagle is moving. Using state-space modeling, New can use the data on the bird’s speed and what researchers already know about eagles’ habits to model how often they might be eating, resting and foraging.
Dolphins and eagles are pretty different. But, New says, when you’re looking at them from a statistical viewpoint, they are much the same. “The statistics that we’re using underneath them to understand the effects of human actions on those species is very, very similar.”
But biology isn’t the only place where statisticians shine. They can work in forensics, social science, public health, sports analytics and more.
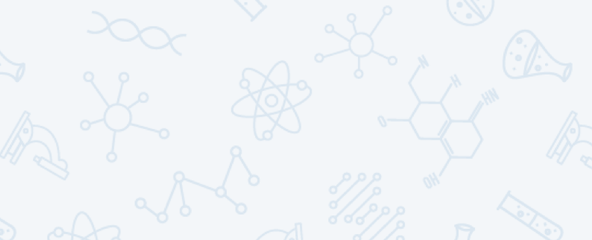
Educators and Parents, Sign Up for The Cheat Sheet
Weekly updates to help you use Science News Explores in the learning environment
Thank you for signing up!
There was a problem signing you up.
Looking for the ‘big picture’
Statisticians can help other researchers make sense of the data they collect, or work on their own. But statistics is also a series of mathematical tools — tools scientists can use to find patterns in the data they collect. Researchers also can use statistics as they think through every step of their studies. These tools help scientists decide how much and what kind of data they will need to gather to answer their research questions. Statistics also help them visualize and analyze their data. Scientists can use this information to put their findings in context.
Statistics can even test how strong connections are. Do they appear to be a fluke or do they point to one thing causing another?
You might wear a yellow jacket every day for a week. And it might also rain every day that week. So there is a link between you wearing a yellow jacket and rainy weather. But did it rain because you wore the yellow jacket? No.
Researchers need to make sure they don’t draw such a false conclusion from what is merely a coincidence. In statistics, this idea can be summed up by the phrase: “Correlation doesn’t imply causation.” Correlation means that two (or more) things are found together or there appears to be some link between them. Causation means that one thing made another thing happen. Statistics can help scientists tell the difference.
What are the chances?
Statisticians evaluate connections in their data by calculating how likely it is that something they observe could be due to chance or error. For instance, researchers might want to know if boat noises affect where whales go in the ocean. They might compare the number of whales in an area with a lot of boats to those in an area with few boats.
But there are many things that can introduce error, here. Both boats and whales move around. Boats make many types of noise. Areas of the ocean might differ in temperature and predators and whale food. Each of these could add error to the measurements scientists take. If enough errors stack up, researchers could come to the wrong conclusion.
A hypothesis is an idea that can be tested. One might be that if a group of whales is exposed to at least 50 hours of human-made noise every year, then their population will decline by at least 10 percent within five years. Scientists could then collect data to test that. Instead, statisticians tend to start with what they call a null hypothesis.It’s the idea that “in whatever relationship you’re exploring, there’s nothing going on,” explains Allison Theobold. She’s a statistician at California Polytechnic State University in San Luis Obispo.
For instance, if New wanted to test the effect of noise on whales, she and her colleagues might count the young born to females exposed to noise. They would be gathering evidence to test if the null hypothesis — that there is no relationship between boat noise and whale visits — is true. If data offer strong evidence against the null hypothesis, then they can conclude there is a relationship between the noise and whale visits.
Scientists also want to make sure they study enough of what they are focusing on. Sometimes known as “n” (for number), a sample size is how many of something researchers study. In the example above, it could be the number of individual whales or whale pods.
If the sample size is too small, researchers won’t be able to draw reliable conclusions. New probably wouldn’t study just two whales. Those two whales could have reactions unlike those of any other whales. New would need to study many whales to find out.
But big sample sizes aren’t always the answer either. Looking at too broad of a group could make the results murky. Maybe a study looked at whales spanning too broad an age range. Here, many could be too young to have babies yet.
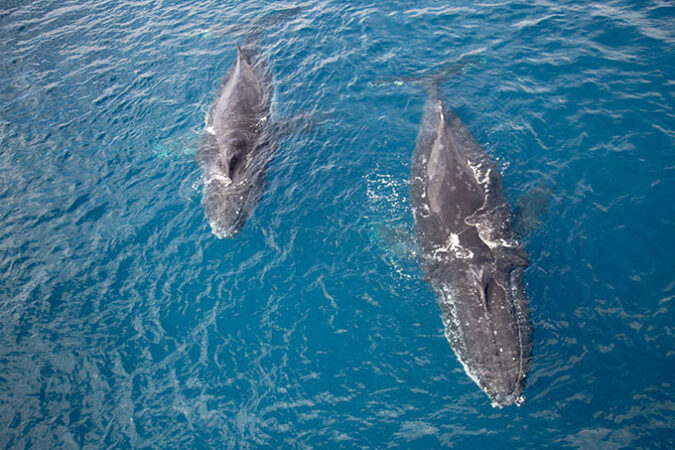
What is statistical significance?
In everyday language, when we say that something is significant, we usually mean that it’s important. But to researchers, being statistically significant means something else: that a finding or conclusion is not likely due to random chance or error.
Researchers often refer to a p-value to decide whether something is statistically significant. Many only consider results statistically significant if the p-value is small. The cutoff commonly used is 0.05 (written p < 0.05). That means that there is a less than a five percent (or 1 in 20) chance that researchers will conclude a relationship is present, when the connection they’re seeing is really due to chance, error or some natural variation in the magnitude of what they’re studying.
But there are problems with using p-values to decide whether findings are important, adds Theobold. In fact, she calls statistical significance the “s word.”
It’s too easy for people to confuse statistical significance with importance, she explains. When Theobold reads a news article that says a study’s finding was statistically significant, she knows that means the researchers “probably got a really small p-value.”
But just because a difference was real doesn’t necessarily mean the difference also was important. It does not even mean the difference was a big one.
Statistical significance may lead some people to pay more attention to studies just because their p-values are small. Meanwhile, studies that could be important may be ignored because their p-values weren’t small enough. Lack of statistical significance does not mean the data were bad or carelessly collected.
Many statisticians — including Theobold — are calling for alternatives to p-values and statistical significance. Effect size is one measure they might use. Effect size tells researchers how strong two things might be linked. For instance, a lot of ocean noise could be associated with 75 percent fewer baby whales being born. That would be a large effect of noise on the number of baby whales. But if that noise only correlated with five percent fewer whales, then the effect size is far smaller.
Statistics may seem like a foreign or even scary word, but it’s used to assess the data behind the coolest studies in STEM. There’s a place for you in stats regardless of whether you’re a natural at math or science, says New.
“I was in remedial math throughout elementary school,” she notes. Yet she ended up with a Ph.D. in statistics. “So it’s not that I was always naturally brilliant at math and stats and then somehow took that to study animals. It’s that I had an interest [in animals] and because I was interested, I was able to overcome what was more challenging for me.”