To ‘green’ AI, scientists are making it less resource-hungry
Curbing AI’s use of energy and water could seriously lessen its threat to our climate
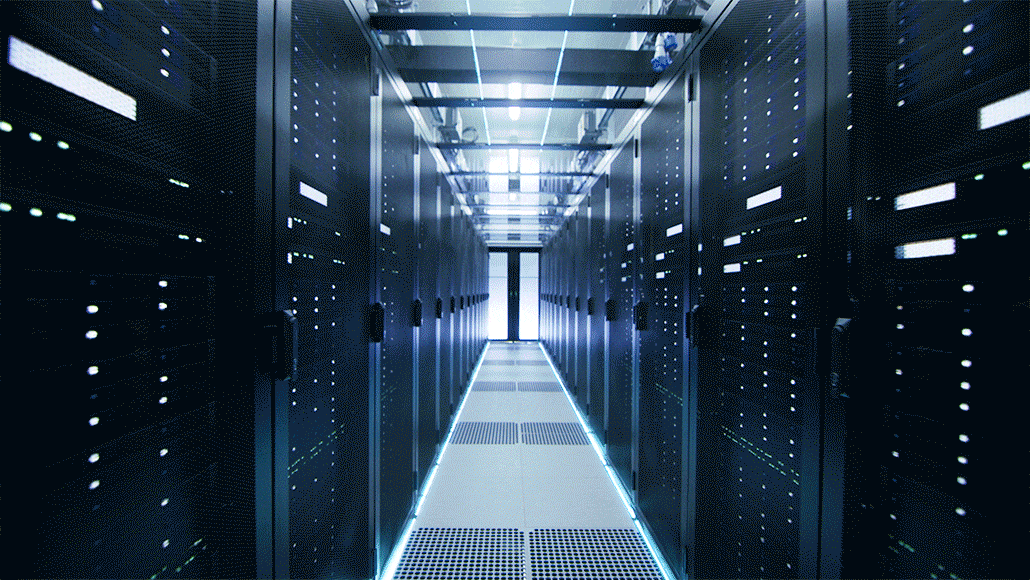
Data centers are the buildings where computers do all the work it takes to keep our digital lives running. They consume around one to two percent of the world’s electricity, according to the International Energy Agency.
gorodenkoff/Creatas Video+/Getty Images Plus
This is the first in a year-long series of stories identifying how the burgeoning use of artificial intelligence is impacting our lives — and ways we can work to make those impacts as beneficial as possible.
Computing equipment stacked all the way to the ceiling. Thousands of little fans whirring. Colored lights flashing. Sweltering hot aisles alongside cooler lanes. Welcome to a modern data center.
Every ChatGPT conversation, every Google search, every TikTok video makes its way to you through a place like this.
“You have to go in with a jacket and shorts,” says Vijay Gadepally with the Massachusetts Institute of Technology, or MIT. As a computer scientist at MIT’s Lincoln Laboratory in Lexington, Mass., he helps run a data center that’s located a couple of hours away by car in Holyoke. It focuses on supercomputing. This technology uses many powerful computers to perform complex calculations.
Entering the data center, you walk past a power room where transformers distribute electricity to the supercomputers. You hear “a humming,” Gadepally says. It’s the sound of the data center chowing down on energy.
Data centers like this are very hungry for electricity and their appetites are growing. Most are also very thirsty. Cooling their hardworking computers often takes loads of fresh water.
More people than ever before are using applications that rely on supercomputers, says Gadepally. On top of that, he adds, supercomputers are doing more energy-intensive things. Stuff like running ChatGPT. It’s an artificial intelligence, or AI, model that can generate code, write text or answer questions. Some scientists estimate that answering a question with ChatGPT or a similar AI tool consumes about 10 times as much electricity as a Google search.
Just two months after it launched, ChatGPT reached 100 million active users, making it the fastest growing app ever. And, Gadepally adds, energy-hungry AI doesn’t just power chatbots. “AI is making its way into everything.” Generating one image using an AI model such as Stable Diffusion can draw as much energy as fully charging a smartphone. That’s the recent finding of researchers at a collaborative AI platform called Hugging Face.
Meanwhile, the climate crisis is worsening. Since people still burn fossil fuels to produce most of our electricity, a growing demand for energy leads to higher releases of greenhouse gases. That’s got some experts looking at how to cut the climate impact of AI. Their goal: to make such increasingly popular AI tools more sustainable.
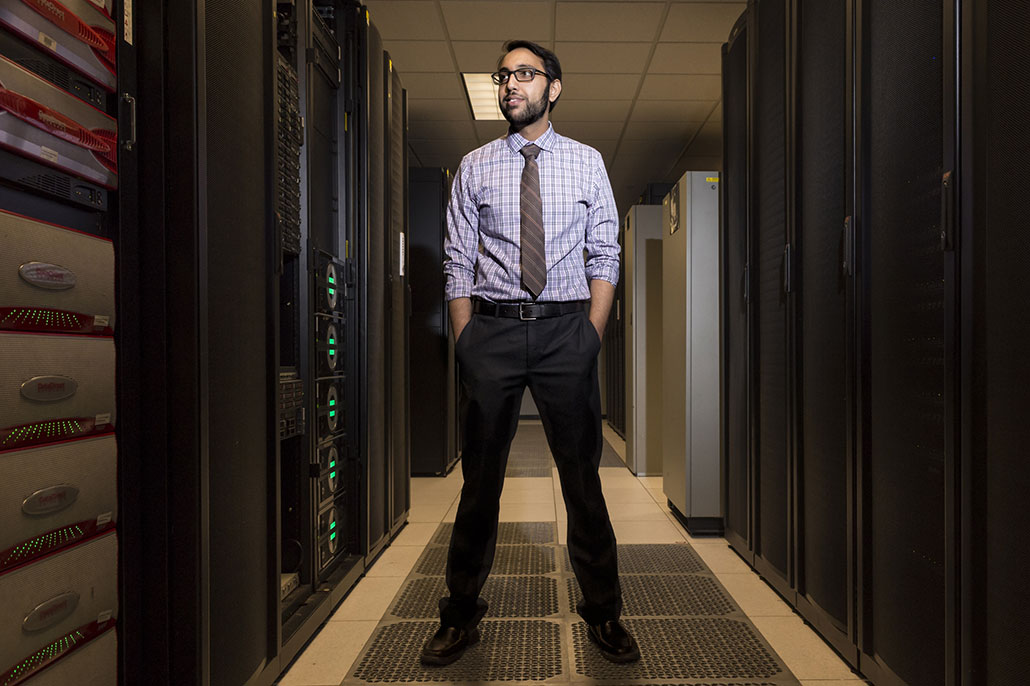
Bigger isn’t always better
AI’s appetite for energy depends on what type of model it is. Many of the ones used in scientific research are quite small. “A lot of the models I’ve trained take a few hours on a personal computer,” says Alex Hernandez-Garcia. This AI expert works as a researcher at Mila, an AI institute in Montreal, Canada. A lean model like that has a teeny-tiny carbon footprint, he says. It may be similar to the power used to keep an incandescent light bulb lit for a few hours.
However, tools like ChatGPT rely on large language models, or LLMs. An LLM is a type of AI based on machine learning. It learns to predict the order of words.
As their name implies, LLMs are big. Really big. Because there is so much language data available to feed them, they tend to be the largest of all machine-learning models. It takes months and many supercomputers to train them, says Hernandez-Garcia.
In a 2023 paper, his team surveyed the carbon footprints of many AI models. Based on this research, he estimated the climate impact of training the LLM GPT-3. (Updated versions of this model run ChatGPT today). Its impact might equal some 450 commercial airplane flights between London and New York City, he found. This research also looked at models trained to classify images, detect objects, translate languages and more.
Making any of these models bigger often provides better results. But a large jump in model size usually leads to only a very tiny increase in its ability, notes Hernandez-Garcia. Bigger isn’t always better, his team has shown. Models whose use led to the most greenhouse-gas emissions didn’t always perform the best, their analysis showed.
In a 2021 paper, Emily M. Bender argued that, in fact, LLMs may be getting too big. Bender is a computational linguist at the University of Washington in Seattle. “AI is a luxury,” she says. Therefore, people should think carefully about the ethics of building ever-larger models.
The worst-case scenario
One measure of an AI model’s size is the number of parameters it contains. Parameters are what get tweaked as the model learns. The more parameters a model has, the more detail it can learn from data. That often leads to higher accuracy.
GPT-2 — an LLM from 2019 — had 1.5 billion parameters. Just a couple years later, GPT-3.5 was using 175 billion parameters. The free version of ChatGPT runs on that model today. Users who pay for the app now get access to GPT-4, an even more advanced LLM. It’s said to manipulate an estimated 1.7 trillion parameters!
The free version of ChatGPT that was running in early 2023 was the one that consumed about 10 times as much energy per question as Google, says Alex de Vries. He’s a PhD student in economics at Vrije (Public) University Amsterdam in the Netherlands. He’s also the founder of Digiconomist. This company studies the impact of digital trends.
Do you have a science question? We can help!
Submit your question here, and we might answer it an upcoming issue of Science News Explores
In a 2023 study, de Vries estimated that at the height of ChatGPT’s popularity, the app was likely consuming about 564 megawatt hours of electricity per day. That’s roughly equal to the daily energy use of about 19,000 U.S. households. So he decided to do a thought experiment: What if every Google search people are doing right now instead went through an LLM such as ChatGPT? “Google alone would be consuming as much power as Ireland,” he realized.
Will AI tools based on giant, energy-hungry LLMs soon gobble up as much electricity as entire countries? Not overnight.
The good news, de Vries says, is that his thought experiment is “an extreme example.” Most tech companies, he notes, can’t afford to buy that much energy. Plus, data centers don’t have enough supercomputers to support such a huge demand for AI. This type of AI requires special computer chips. Right now, factories can’t make those chips fast enough, he says. “That gives us some time to reflect on what we’re doing” — and maybe do things differently.
Putting data centers on a diet
Gadepally and his team aren’t just reflecting — they’re acting. They’ve found several ways to put their data center on an energy diet.
Not all AI tasks require a humongous energy hog, the Hugging Face study showed. These researchers measured the carbon footprint of small models trained only to perform a single task, such as tagging movie reviews as either positive or negative. The footprint of tagging 1,000 reviews with a small model was around 0.3 gram of carbon dioxide, or CO2. When the researchers did the same task with big, powerful LLMs, they found emissions of around 10 grams of CO2 — 30 times as much.
Gadepally’s team has developed a new AI model that could help rein in other AI models. Called CLOVER, it figures out what a user is trying to do, then selects only as big a model as that task truly needs.
CLOVER can “mix and match models to best suit the task at hand,” says Gadepally. This year, his team reported that CLOVER can cut the greenhouse-gas emissions of AI use at a data center by more than 75 percent. With those savings, the accuracy of the results that AI models provide drops by only 2 to 4 percent.
Video games provided the idea for another energy-saving trick. “One of our colleagues is a big gamer,” notes Gadepally. Machine-learning models run on what’s known as graphics processing units, or GPUs. High-end video games use this same type of computer chip. His colleague found he could put a brake on the power his GPU could draw while playing games. Scientists refer to this tactic as “power capping.” Usually, it does not impact the quality of games running on GPUs.
As GPUs work harder, they draw more power — and heat up. If they aren’t allowed to draw as much power at once, their work may take a bit longer. But power-capped GPUs aren’t wasting energy ramping up and then slowing back down, the way non-capped GPUs do. Plus, power-capped GPUs don’t get as hot. That means they also don’t need as much cooling.
Gadepally’s team tested this with an LLM named BERT. Without power-capping, it took 79 hours to train BERT. With power-capping, training took three hours more. But they saved energy, he says, equal about to what’s used in a week by the average U.S. household. That’s a big energy savings for a small amount of added time.
Their tests were so successful that they’re now using power-capping throughout the data center. “Some people have said we’re a bit weird for doing it,” says Gadepally. But he hopes others will follow their lead.
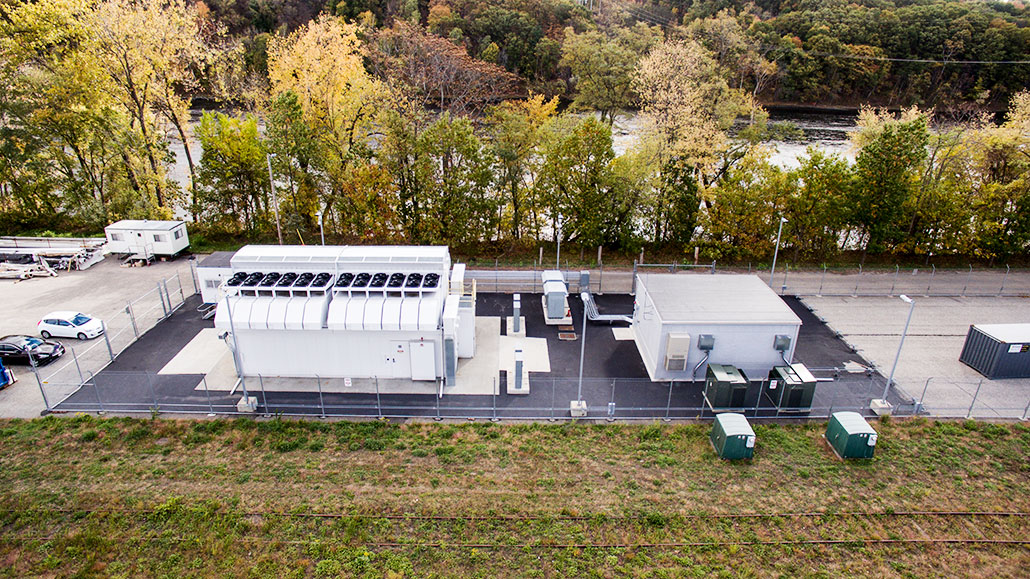
How to ‘imagine AI differently’
The data center where Gadepally’s group did all these tests actually has a fairly small carbon footprint. That’s because its electricity mainly comes from a nearby hydroelectric dam. This is a water-powered energy source that doesn’t release much greenhouse gas into the air. Tech companies can reduce their climate impact by building data centers or scheduling data calculations at places that get most of their power from renewable sources.
However, there’s only so much green energy to go around. Using it for AI means not using it for something else.
Also, the best places to collect green energy may not be ideal for data centers. Arizona is a state where a lot of solar and wind farms already feed electricity into the power grid. The state’s weather, however, is very hot. Data centers everywhere need to keep their computers from overheating. Most use fresh water to do this.
“Computing needs a tremendous amount of water,” points out Shaolei Ren. He’s a computer engineer at the University of California, Riverside. Climate change is making fresh water scarcer, especially in places like Arizona. So thirsty data centers built in those areas can become a big problem.
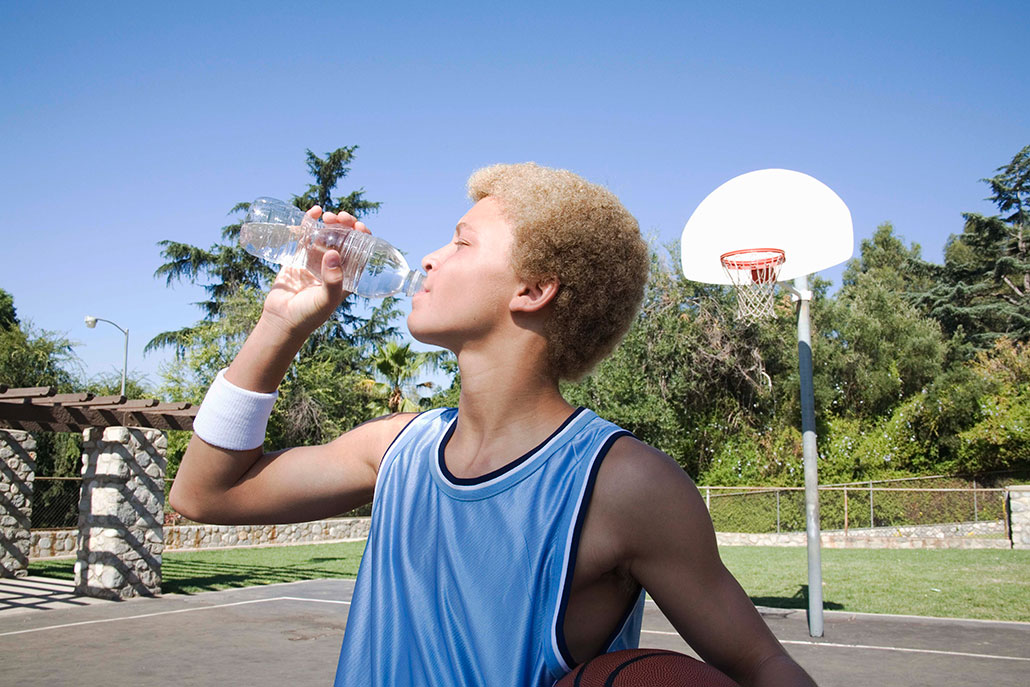
Hernandez-Garcia, Ren and other experts have called for tech companies to measure and report on their greenhouse-gas emissions and water footprints. That’s a great idea. But there’s only so much that tech companies can do to cut these impacts while building ever-larger AI models.
Real change starts deeper, with the way society approaches the systems it builds, suggests Priya Donti. Before throwing all available resources into a system, we need to consider that system’s sustainability as well as its environmental and social impact. Donti is a computer scientist at MIT in Cambridge, Mass. She also co-founded the organization Climate Change AI. This group looks at ways AI and machine learning can help society reach climate goals.
Right now, says Donti, large tech companies are driving the emergence of ever bigger AI models. But “it doesn’t have to be that way,” she says.
Researchers are finding creative ways to make smart, useful, greener AI. For example, they can transfer insights between AI models. They also can train using less — but higher quality — data.
One company, Numenta, is looking to the human brain for inspiration. Designing AI models that are more similar to the brain means “much less math has to be done,” explains co-founder Jeff Hawkins. And fewer calculations means a lower demand for energy.
“AI doesn’t have to be super, super data-hungry or super, super compute-hungry,” says Donti. Instead, we can “imagine AI differently.”
This is another in our series of stories identifying new technologies and actions that can slow climate change, reduce its impacts or help communities cope with a rapidly changing world.