Scientists enlist computers to hunt down fake news
And these automatons tend to outperform people at scouting out bogus claims
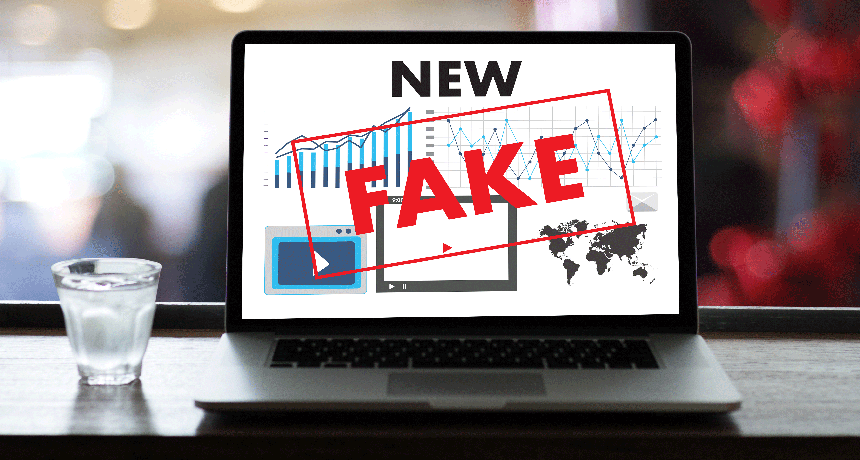
Some lies are easy to spot. Take that report that First Lady Melania Trump wanted an exorcist to cleanse the White House of Obama-era demons. (Bogus!) Then there was that piece on an Ohio school principal who was arrested for defecating in front of a student assembly. (Also untrue.) Sometimes, however, fiction blends a little too well with fact. For instance, did cops really find a meth lab inside an Alabama Walmart? (Again, no.)
We live in a golden age of misinformation. So anyone scrolling through a slew of stories might easily be fooled. In fact, data show, plenty of us are.
On Twitter, falsehoods spread further and faster than the truth, a March 9 study found. Online bots have been accused of spreading these tall tales. But the study in Science found that people shared more bogus tales than did web bots.
Plenty of fake-news stories moved around the web in the weeks leading up to the 2016 U.S. presidential election, U.S. intelligence officials reported this year. And the most popular of these fake news reports got more Facebook shares, reactions and comments than did truthful news, according to one analysis in BuzzFeed News.
Luca de Alfaro is a computer scientist at the University of California, Santa Cruz. In the old days, he says, “you could not have a person sitting in an attic and generating conspiracy theories at a mass scale.” Then along came the internet, and more recently social media. Now, peddling lies is truly easy — especially as the internet can essentially hide who is behind a post. Consider that group of teens in the European nation of Macedonia, two years ago. They raked in huge sums of cash by writing popular fake news on topics that were popular during the U.S. presidential election. Readers thought those posts had been written by true journalists.
Most web users probably don’t post bunk on purpose. They likely “share” posts they simply didn’t take the time to fact-check. And that’s easy to do if you’re suffering from information overload. Or if you are overworked or tired.
Confirmation bias — already suspecting an issue is true, or not — can make the problem worse. Consider reports from sources you don’t recognize. “It’s likely that people will choose something that conforms to their own thinking,” says Fabiana Zollo. That’s true “even if that information is false,” says this computer scientist. She works in Italy at Ca’ Foscari University of Venice. Her studies focus on how information circulates within social networks.
Fake news doesn’t just threaten the integrity of elections. It also can erode the trust people place in real news. Lies posing as truth can even threaten lives. False rumors in India, earlier this year, incited lynchings. More than a dozen people died. And the source of those rumors: posts on WhatsApp, a smartphone messaging system.
To help sort fake news from truth, scientists have begun building new computer programs. They sift through online news stories, looking for telltale signs of fake facts or claims. Such a program might consider how an article reads. It might also look at how readers respond to the story on social media. If a computer spied a possible lie, its job would be to alert human fact-checkers. They would then be asked to make any final judgment call.
Giovanni Luca Ciampaglia works at Indiana University in Bloomington. Today’s automatic lie-detection tools are “still in their infancy,” he says. This means there’s still a lot of work to be done before many fact-checking programs are let loose on the internet.
The good news: Research teams around the world are forging ahead. They realize that the internet is a fire hose of facts or purported facts. So human fact-checkers clearly need help. And computers are beginning to step up to the challenge.
Reader referrals
Visitors to real news websites mainly reach those sites directly or from search engines, such as Google or Bing. Fake news sites attract a much higher share of their incoming web traffic through links on social media.
Substance and style
When inspecting a news story, there are two major ways to tell if it looks like a fraud. The first: What is the author saying? Then look at how the author phrases it.
Ciampaglia and his colleagues have just built a system to automate this task. It checks how closely related a statement’s subject and object are. To do this, the computer sifts through a vast network of nouns. This network has been built from facts found in the “infobox” on the right side of every Wikipedia page. (Related networks have been built from other reservoirs of knowledge, such as research databases.)
The computer judges two nouns — that subject and object — as being “connected” if each appears in the infobox of the other. The computer then measures how closely a statement’s subject and object are linked within this network. The closer they are, the more likely the computer program is to label a statement as true.
Take the false assertion: “Barack Obama is a Muslim.”
There are seven degrees of separation between “Obama” and “Islam” in the noun network. These include very general nouns, such as “Canada,” that link to many other words. Given this, the computer program decided Obama was unlikely to be Muslim.
The Indiana University team unveiled this computer fact-checker, three years ago, in PLOS ONE.
Roundabout route
An automated fact-checker judges the assertion “Barack Obama is a Muslim” by studying degrees of separation between the words “Obama” and “Islam” in a noun network built from Wikipedia info. The very loose connection between these two nouns suggests the statement is false.
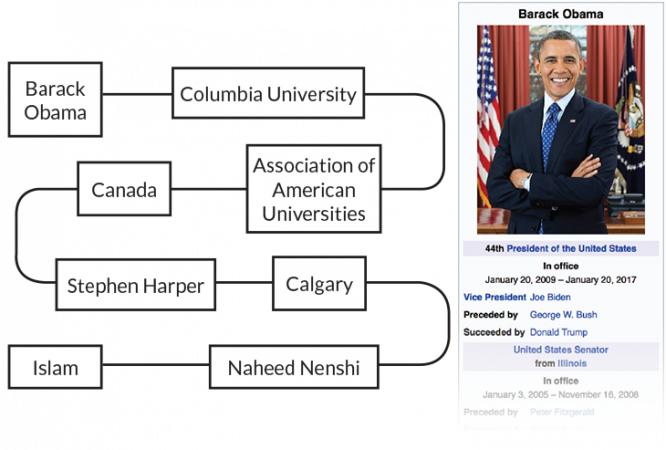
But looking to gauge the truth of statements based on how closely the subjects and objects are linked has its limits. For instance, the system found it likely that former President George W. Bush was married to Laura Bush. Great. It also decided he was probably married to Barbara Bush, his mother. Not so great. The Indiana group is now working to improve its program’s skill in judging how nouns in the network link to one another.
Grammar checks
Writing style may also point to untruths.
Benjamin Horne and Sibel Adali are computer scientists at Rensselaer Polytechnic Institute in Troy, N.Y. They analyzed 75 true articles from news outlets deemed very trustworthy. They also probed 75 false stories from websites accused of dispensing untrustworthy info. Compared with truthful news, bogus stories tended to be shorter. They also were more repetitive. They contained more adverbs (such as quite, always, never, incredibly, highly, unbelievably, absolutely, basically). Fake stories also had fewer quotes, fewer technical words and fewer nouns.
The researchers used these observations to make a computerized lie detector. It focused on the number of nouns, quotes, redundancies and words in a story. And it correctly sorted fake news from true 71 percent of the time. (For comparison, a random sorting of fake news from true should be right about 50 percent of the time).
Truth and lies
A study of hundreds of articles revealed differences in style between genuine and made-up news. Real stories contained more language conveying differentiation. False stories expressed more certainty.
Frequently used words in legitimate news | Frequently used words in fake news | ||
---|---|---|---|
“think” “know” “consider” |
Words that express insight | Words that express certainty | “always” “never” “proven” |
“work” “class” “boss” |
Work-related words | Social words | “talk” “us” “friend” |
“not” “without” “don’t” |
Negations | Words that express positive emotions | “happy” “pretty” “good” |
“but” “instead” “against” |
Words that express differentiation | Words related to cognitive processes | “cause” “know” “ought” |
“percent” “majority” “part” |
Words that quantify | Words that focus on the future | “will” “gonna” “soon” |
Horne and Adali unveiled their system at the 2017 International Conference on Web and Social Media in Montreal, Canada. The two are now looking for ways to boost the system’s accuracy.
Computer scientist and engineer Vagelis Papalexakis works at the University of California, Riverside. His team also built a fake news detector. In one test, it sorted through roughly 64,000 articles that had been shared on Twitter. Half were fake stories. The scientists then directed the computer to sort these stories into groups based on how similar they were. The researchers didn’t instruct their computer on how to judge what was “similar.” Now the researchers identified 5 percent of the stories for the computer as being true or bogus.
Fed just that little bit of information, the program was able to predict which of the rest of the stories were true or not. And it was right for almost seven in every 10 of the other stories. The researchers described their work April 24 at arXiv.org.
Adult supervision
Getting it right about 70 percent of the time isn’t nearly accurate enough to trust. But it might be good enough to offer a proceed-with-caution alert when a reader opens up a suspicious story on the web.
In a similar kind of first step, social-media sites could use computer watchdogs to prowl news feeds for questionable stories. Those that get flagged this way could then be sent to human fact-checkers for a closer look.
Today, Facebook considers feedback from users as it decides which stories to fact-check. Those comments might point to skeptical claims or clear falsehoods. The media company then sends these stories to be reviewed by professional skeptics (such as FactCheck.org, PolitiFact or Snopes). And Facebook is open to using other signs to rout possible hoaxes, notes its spokesperson Lauren Svensson.
No matter how good computers get at finding fake news, they shouldn’t totally replace people in fact-checking, Horne says. The final judgment may require a better understanding than today’s computers have for the topics being discussed, for the language or for the culture.
Alex Kasprak is a science writer at Snopes. It’s the world’s oldest and largest online fact-checking site. He imagines a future where fact-checking will be like computer-assisted voice transcription. The first, rough draft of a transcription will attempt to identify what was said. A human still has to review that text, however. Her role: looking for missed details, such as spelling errors or words the program just got wrong. Similarly, computers could compile lists of suspect articles for people to check, Kasprak says. Here, people would then be asked to offer the final assessment of what reads true.
Eyes on the audience
Even as computers get better at flagging bogus stories, there’s no guarantee that fake news creators won’t step up their game to stay just ahead of the truth cops. If computer programs are designed to be skeptical of stories that are overly positive or express lots of certainty, then authors could craft their lies to read that way.
“Fake news, like a virus, can evolve and update itself,” says Daqing Li. He’s a network scientist at Beihang University in Beijing. And he has studied fake news on Twitter. Fortunately, online news stories can be judged on more than their content. And some of these — such as the posts people make about these stories on social media — might be much harder to mask or alter.
For instance, tweets about a certain piece of news can sometimes be good clues to whether a story is true, notes Juan Cao. She’s a computer scientist in Beijing at the Institute of Computing Technology. (It’s part of the Chinese Academy of Sciences.)
Sheeples
The majority of Twitter users who discussed false rumors about two disasters posted tweets that just spread these rumors. Only a small fraction had looked for verification that a story was real or expressed doubt about the stories.
Cao’s team built a system that could round up the tweets posted on Sina Weibo. That’s China’s version of Twitter. The computer focused on tweets mentioning a particular news event. It then sorted those posts into two groups: tweets that expressed support for the story and those that opposed it. The system then considered several factors to judge how truthful the posts were likely to be. If, for example, the story centered on a local event, the user’s input was seen as more credible than a comment from someone far away. The computer also judged as suspicious the frequent tweets on a story by someone who had not tweeted for a long time. It used other factors, too, to sift through tweets.
Cao’s group tested their system on 73 real and 73 fake stories. (They had been labeled as such by organizations such as Xinhua, China’s state-run News Agency.) The computer sifted through about 50,000 tweets about these stories. It correctly labeled 84 percent of the fakes. Cao’s team described its findings in 2016 at an artificial intelligence conference held in Phoenix, Ariz.
The U.C. Santa Cruz team similarly showed that hoaxes can be picked out of real news based on which users “liked” these stories on Facebook. They reported the finding, last year, at a machine-learning conference in Europe.
A computer might even look at how the story is getting passed around on social media. Li at Beihang University and his colleagues studied the shape of the networks that branch out from news stories on social media. The researchers reviewed reposted stories and the networks of readers that they involved for some 1,700 fake and 500 true news stories on Weibo. They did the same for about 30 fake and 30 true news networks on Twitter. On both social-media sites, Li’s team found, most people tend to repost real news straight from a single source. The same was not true for fake news. These stories tended to spread more through people reposting from other reposters.
A typical network of reposted truthful stories “looks much more like a star,” Li says. “Fake news spreads more like a tree.” Li’s team reported its new findings March 9 at arXiv.org. They suggest that computers could analyze how people engage with social media posts as a test for truthfulness. Here, they wouldn’t even have to put individual posts under the microscope.
Branching out
On Twitter, most people reposting real news (red dots) get it from a single, central source (green dot). Fake news, in contrast, spreads more through people reposting from other reposters.
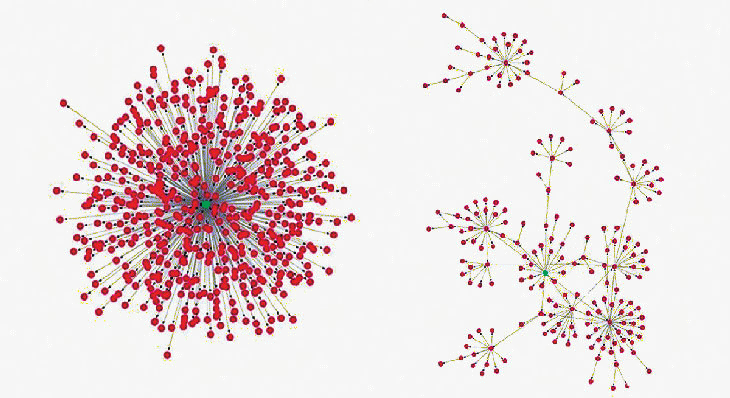
Truth to the people
What’s the best way to deal with lies on social media networks? Right now, no one really knows. Just erasing bogus articles from news feeds is probably not the way to go. Social media sites that exert such control over what visitors see would be viewed with alarm. It’s similar to what people who live under dictators encounter, notes Murphy Choy. He’s a data analyst at SSON Analytics in Singapore.
Internet sites could put warning signs on stories they suspect of being untrue. But labeling such stories may have an unfortunate effect. It may cause people to think that the remaining unlabeled stories are all true. In fact, they may simply have not been reviewed by a fact-checker. This was the conclusion, anyway, of research posted last year on the Social Science Research Network. Gordon Pennycook in Canada at the University of Regina, and David Rand of Yale University in New Haven, Conn., conducted the study.
Facebook has taken a different approach. It shows debunked stories lower in a user’s news feeds. And that can cut a false article’s future views by as much as 80 percent, company spokesperson Svensson says. Facebook also displays articles that debunk false stories whenever users encounter the related stories. That technique, however, may backfire. Zollo in Venice, Italy, works with Walter Quattrociocchi. They conducted a study of Facebook users who like and share conspiracy news. And after these readers interacted with debunking articles, they actually increased their activity on Facebook’s conspiracy pages, they reported this past June.
There’s still a long way to go in teaching computers — and people — to reliably spot fake news. As the old saying goes: A lie can get halfway around the world before the truth has put on its shoes. But keen-eyed computer programs may at least slow down fake stories with some new ankle weights.