The brain of a tiny worm inspired a new type of AI
A liquid neural network is smaller and more adaptable than mainstream AI
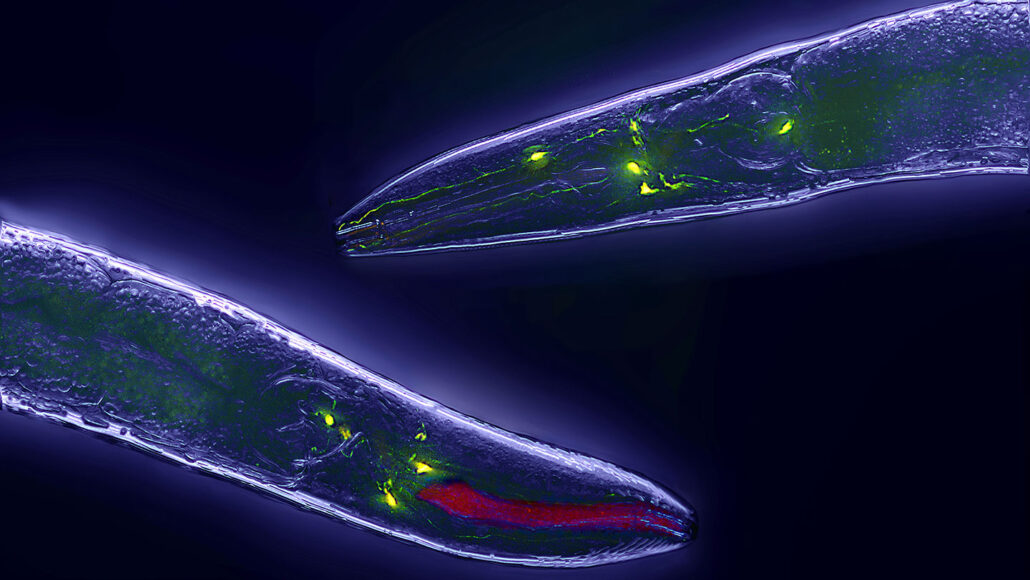
C. elegans, seen here, is a tiny, transparent roundworm. At 1 millimeter (four 1/100ths of an inch) long, it could fit on the tip of a pencil. Its brain is even tinier. Yet even its simple brain does a far better job at learning and adapting to the environment than any AI system people use today.
HeitiPaves/iStock/Getty Images Plus
This is another in a year-long series of stories identifying how the burgeoning use of artificial intelligence is impacting our lives — and ways we can work to make those impacts as beneficial as possible.
Ramin Hasani peered through a microscope at a tiny, wriggling worm. Fascinating, he thought. Later, he would say, “It can move better than any robotic system that we have.”
This tiny worm has an even tinier brain, yet it can move and explore with ease. Its brain has now inspired Hasani and his team to develop a new type of artificial intelligence.
They call it a liquid neural network.
In both the worm’s brain and your big human brain, cells called neurons link to each other through connections called synapses. These form interconnected networks that process thoughts and sensations.
ChatGPT and most of today’s popular AI models run on artificial neural networks, or ANNs. Despite their name, though, these networks have almost nothing in common with a true brain.
Liquid neural networks are a new type of ANN. They more closely model our brains. “I think Ramin Hasani’s approach represents a significant step towards more realistic AI,” says Kanaka Rajan. She was not involved with the new work. But she knows about such things. She’s a computational neuroscientist at Harvard Medical School and the Kempner Institute for the Study of Natural and Artificial Intelligence in Boston, Mass. She uses AI to better understand the brain.
Compared to a standard ANN, a liquid neural network needs less energy and computer power to run. Yet it can solve some problems more nimbly.
Hasani’s team at the Massachusetts Institute of Technology (MIT), in Cambridge, showed this in tests with self-driving cars and drones. And in December 2023, his group launched a company to bring the technology into the mainstream. Hasani is now CEO of Liquid AI, also in Cambridge, Mass.
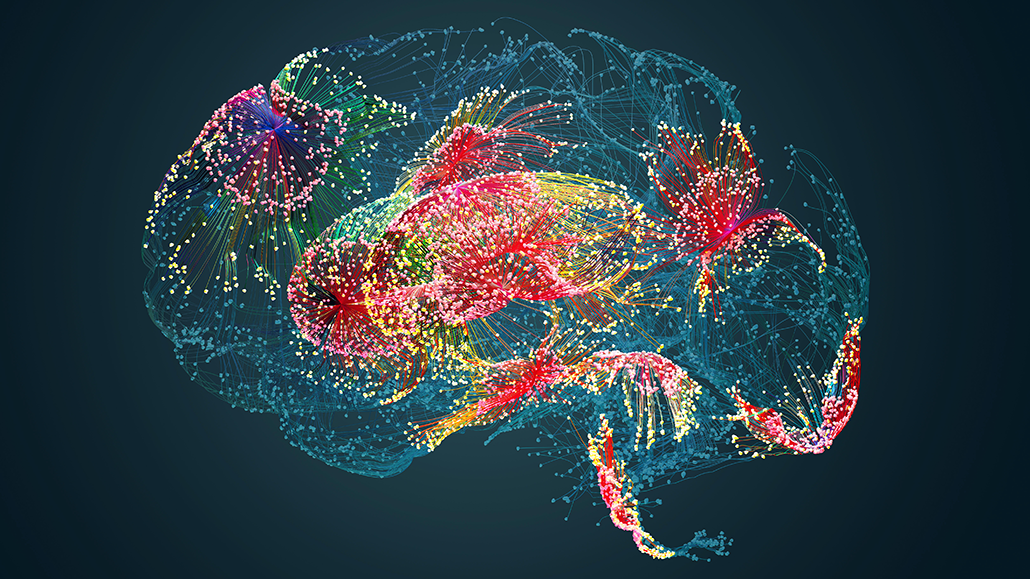
Thinking smaller and smarter
The best ideas strike when you’re in the shower or out for a run, says Daniela Rus. She directs MIT’s Computer Science and Artificial Intelligence Laboratory. The idea for liquid neural networks first emerged one hot summer day several years ago. This co-founder of Liquid AI was at a conference. Ramin Hasani’s PhD advisor, Radu Grosu, was there, too. The pair went for a run and talked about their work.
Grosu is a computer scientist at the Technical University of Vienna in Austria. At the time of this conference, he and Hasani were making models of the brain of C. elegans. This tiny worm has just 302 neurons. Some 8,000 connections link them up. (For comparison, the human brain has some 100 billion neurons and 100 trillion connections.)
Rus was working on self-driving cars. To train the car, her team was using an ANN with tens of thousands of artificial neurons and a half million connections.
If a worm doesn’t need very many neurons to get around, Rus realized, maybe AI models could make do with fewer, too. She recruited Hasani and another of Grosu’s students to move to MIT. In 2020, they began a new project — to give a car a more worm-like brain.
At the time, most AI researchers were building bigger and bigger ANNs. Some of the biggest ones today contain hundreds of billions of artificial neurons with trillions of connections! Making these models bigger has tended to make them smarter. But they also got more and more expensive to build and run.
Might it be possible to make ANNs smarter by taking the worm approach — going smaller?
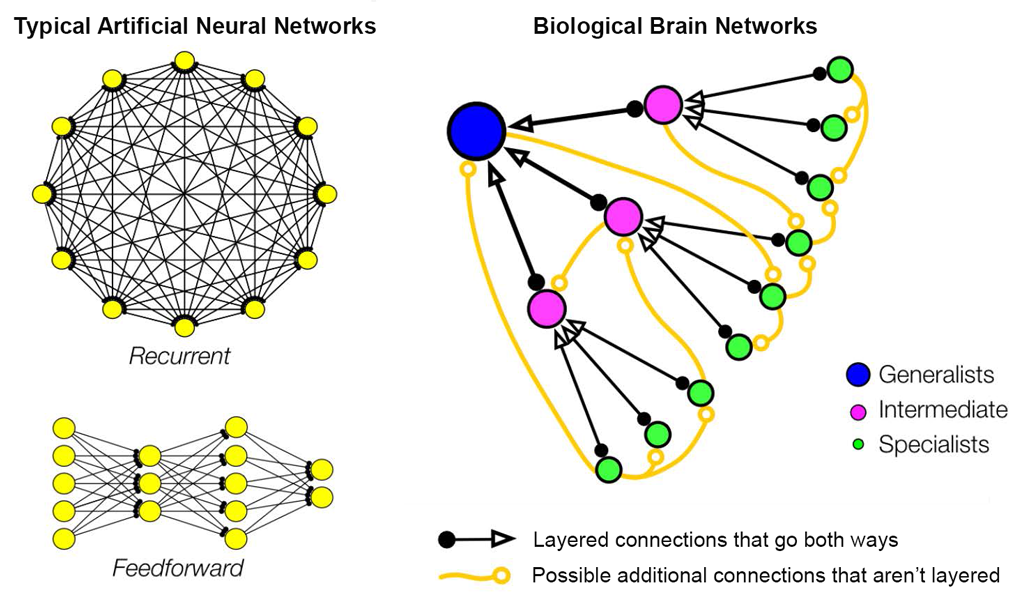
Simplifying the math
Brains, even worm brains, are amazingly complex. Scientists are still working out exactly how they do what they do.
Hasani focused on what is known about how worm neurons influence each other.
Those in C. elegans don’t always react the same way to the same input. There’s a chance — or probability — of different outputs. The timing matters. Also, the neurons pass information through a network both forward and backward. (In contrast, there’s no probability, timing or backward-flowing information in most ANNs.)
It takes some tricky math, called differential equations, to model the more brain-like traits in neurons. Solving these equations means performing a series of complex calculations, step by step. Normally, the solution to each step feeds into the equation for use in the next step.
But Hasani found a way to solve the equations in a single step. Rajan says this is “remarkable.” This feat makes it possible to run liquid neural networks in real time on a car, drone or other device.
Do you have a science question? We can help!
Submit your question here, and we might answer it an upcoming issue of Science News Explores
An ANN learns a task during a period called training. It uses examples of a task to adjust connections between its neurons. For most ANNs, once training is over, “the model stays frozen,” says Rus. Liquid neural networks are different. Even after training, Rus notes, they can “learn and adapt based on the inputs they see.”
In a self-driving car, a liquid neural network with 19 neurons did a better job staying in its lane than the large model Rus had been using before.
Her team studied each system to see what it was paying attention to while driving. The typical ANN looked at the road, but also at lots of bushes and trees. The liquid neural network focuses on the horizon and the edges of the road, “which is how people drive,” Rus says.
She recalls thinking: “We have something special here.”
Go to the red chair
Next, her team tested how adaptable liquid neural networks could be.
To get ready, they made a set of videos. In each, someone piloted a drone toward an object sitting in the woods. They did this during summer, fall and winter. And they used different objects, including a bright red chair.
They then used these videos to train six different types of neural network. Two were liquid neural networks. The training did not explain that the goal was to reach a target object. This is what the team hoped the AI models would figure out on their own.
For the test, the team set up the red chair in a part of the woods that looked a little different from the training videos. All the AI models did well here.
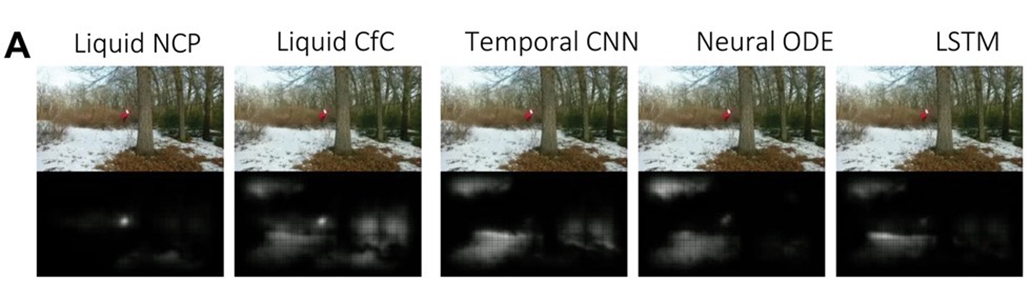
Then they put the chair next to a building. Then they set it on a patio among other chairs. These environments looked completely different from the training videos — and tripped up each typical ANN. “It actually doesn’t know where to look,” said Hasani, speaking at the EmTech Digital conference at MIT this past May. Those other ANNs, he explained, just “didn’t understand what the task was.”
But liquid neural networks usually succeeded.
Next, the team tried putting the red chair much farther away than it was in the training videos. A typical ANN failed every time now. But a liquid neural network? It succeeded nine out of every 10 tries.
How many neurons and connections did it take to do this? “For the drones, we had 34 neurons,” says Rus. A mere 12,000 connections linked them up.
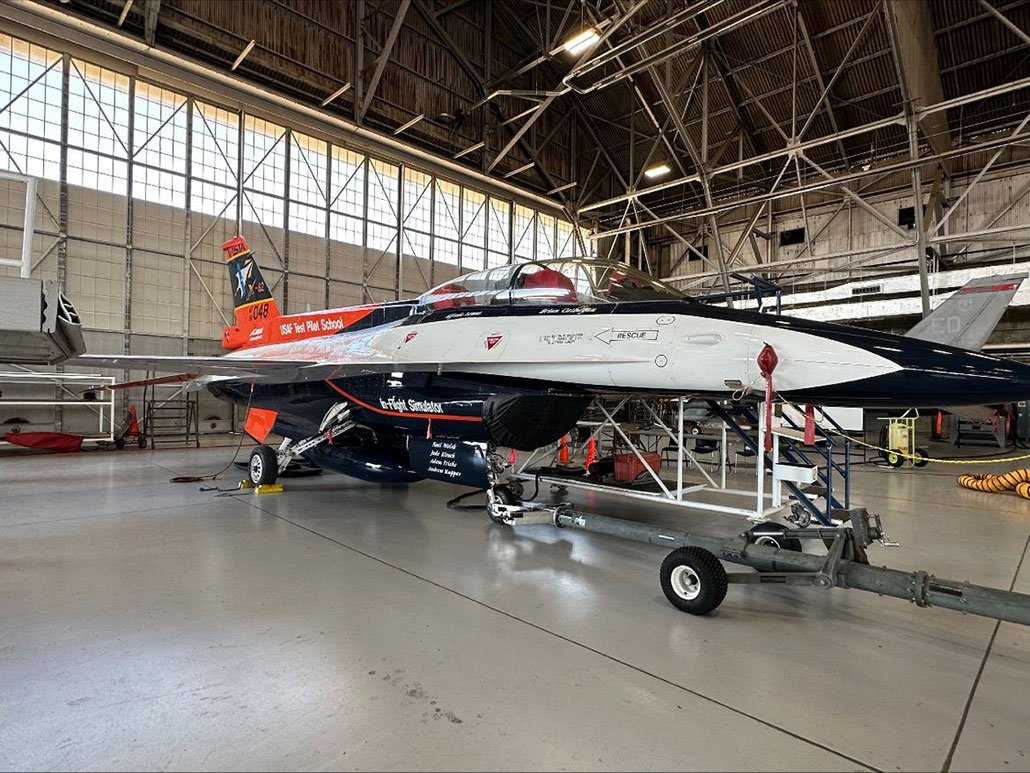
A new flavor of AI
Since then, the team has worked with the U.S. military’s Defense Advanced Research Projects Agency to test the technology in flying an actual aircraft. And Liquid AI has built larger liquid neural networks. Its biggest has 100 billion connections, Hasani said at the MIT conference. This is still far smaller than a state-of-the-art large language model.
“You can think of this like a new flavor of AI,” says Rajan. She notes that being very adaptable has a downside. It “might complicate efforts to understand and control [the model’s] behavior fully.” But she said she would certainly consider using liquid neural networks in her own work to better understand the brain.
“I’m excited about Liquid AI,” says Rus. “I believe it could transform the future of AI and computing.”
And it all started with a tiny worm.
This is one in a series presenting news on technology and innovation, made possible with generous support from the Lemelson Foundation.