Explainer: What is generative AI?
All types of bots are emerging to create images, computer code, articles, ads, songs and more
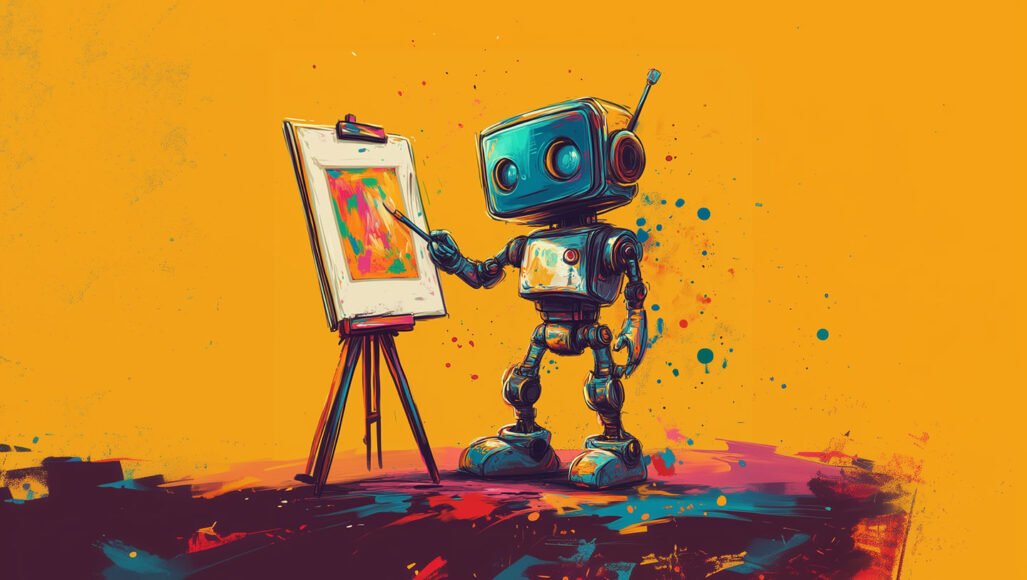
Can robots make art? Sort of. A type of artificial intelligence known as generative AI can produce images. These tools use machine learning to analyze lots of existing images that people have made. The AI models can then use what they “learned” to produce new images, like this one.
K. Hulick/Midjourney
A sweaty football player pours his drink right past his mouth. Then the bottle merges with his nose. This bizarre video opened a 2024 Super Bowl ad. The video wasn’t real. The maker of a sports drink created it using generative artificial intelligence.
Artificial intelligence, or AI, is any technology that can perform tasks that usually require a human brain. Tasks that involve creating something — such as a video, picture, article or song — fall under a special category: generative AI. That Super Bowl ad was making fun of early AI-made content, which tended to come out strangely. The ad said, “There’s no substitute for real.”
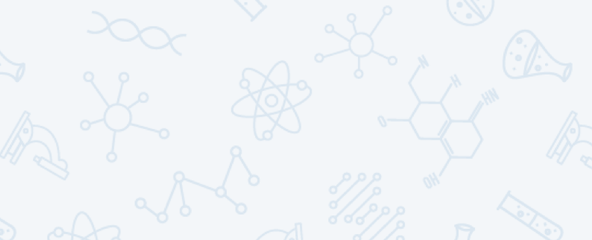
Educators and Parents, Sign Up for The Cheat Sheet
Weekly updates to help you use Science News Explores in the learning environment
Thank you for signing up!
There was a problem signing you up.
Yet AI-generated content is spreading widely. It’s also getting more realistic. Sometimes it seems so genuine it could be mistaken for reality.
People are using generative AI to craft funny social media memes and to help with homework. They also are using it to write computer code, generate quizzes, illustrate books, summarize scientific research and even to help identify potential new medicines.
However, generative AI has been stirring up plenty of controversy.
It sometimes gets facts wrong. It sometimes produces biased content. And some people are using it to cheat or spread misinformation. Plus, many artists and creators are upset, complaining that this new tech exploits or undermines their work.
Like any tech, generative AI has both good and bad sides. Let’s learn how it works.
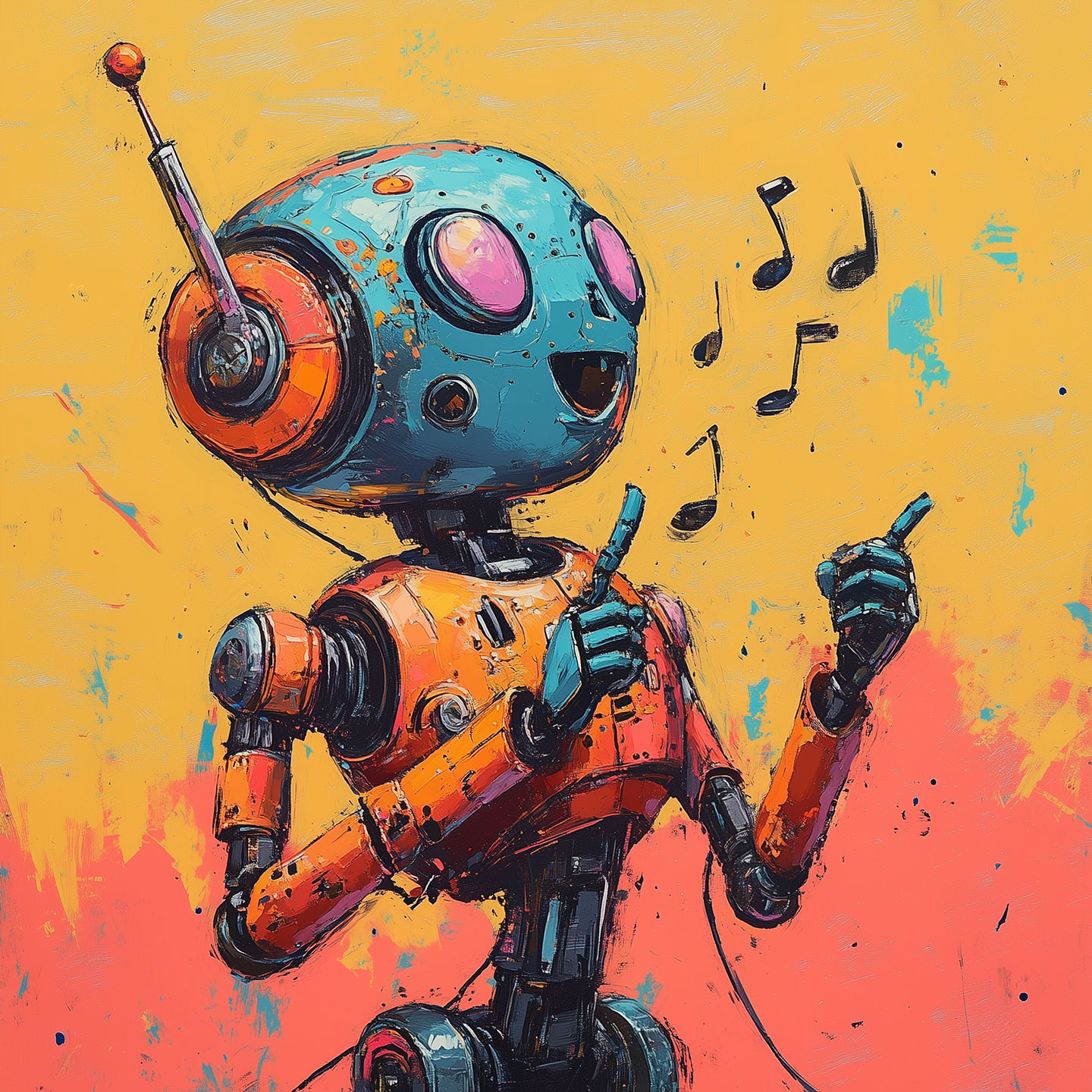
Welcome to the bot zoo
To use generative AI, someone first comes up with a prompt. This may be a question they want answered. Or they might describe an image or video or song they want made. They then give this prompt to an AI model, which is a type of smart computer algorithm.
The model answers the question or creates whatever the person requested. This may take only a few seconds.
In recent years, companies have developed a huge number of tools people can use to interact with generative AI models. These are sometimes called bots.
The Midjourney and Stable Diffusion bots can make artsy or highly realistic images. Sora and Runway create videos. MuseNet and Audiocraft compose music. Chatbots such as ChatGPT and Claude spit out articles, emails, stories, computer code and more. Some of these bots are built on top of foundation models, such as GPT-4 or BERT. These can respond to and generate a mix of different types of content.
Generative AI has been around in some form since at least 1961. That’s when a chatbot named ELIZA was developed that could message back and forth with people. A simple AI model made this possible. It followed rules to select from a list of prewritten responses. Today’s bots are far more complex.
From training to talking
Unlike ELIZA, modern generative AI models don’t follow pre-programmed rules. Instead, they learn from examples during a process called training. (This is true for most types of AI that are popular today, not just generative AI.)
In general, the more examples on which an AI model trains, the better. Pulling together huge sets of data for training is an important part of AI development. To train a self-driving car, for example, developers need lots of driving data.
The most popular generative AI models today trained on staggeringly huge datasets. For example, the image-generating models Stable Diffusion and Midjourney both trained on the same dataset. It contains 2.3 billion images with captions. ChatGPT’s training dataset is not public. But it likely contained a total of around 300 billion words. These mainly came from books, websites and other online content.
During training, most of today’s AI models rely on a technique called deep learning. This is a way of churning through the heap of example data in search of patterns. This process essentially builds a series of maps. Things that often show up together in the data get placed close together in these maps.
Here’s how this works for an AI model that analyzes images. It builds a very low-level map that groups individual pixels. Different maps group patterns of pixels. High-level maps then track image styles.
Similarly, a model learning from music will map everything from individual notes to the genre of a song.
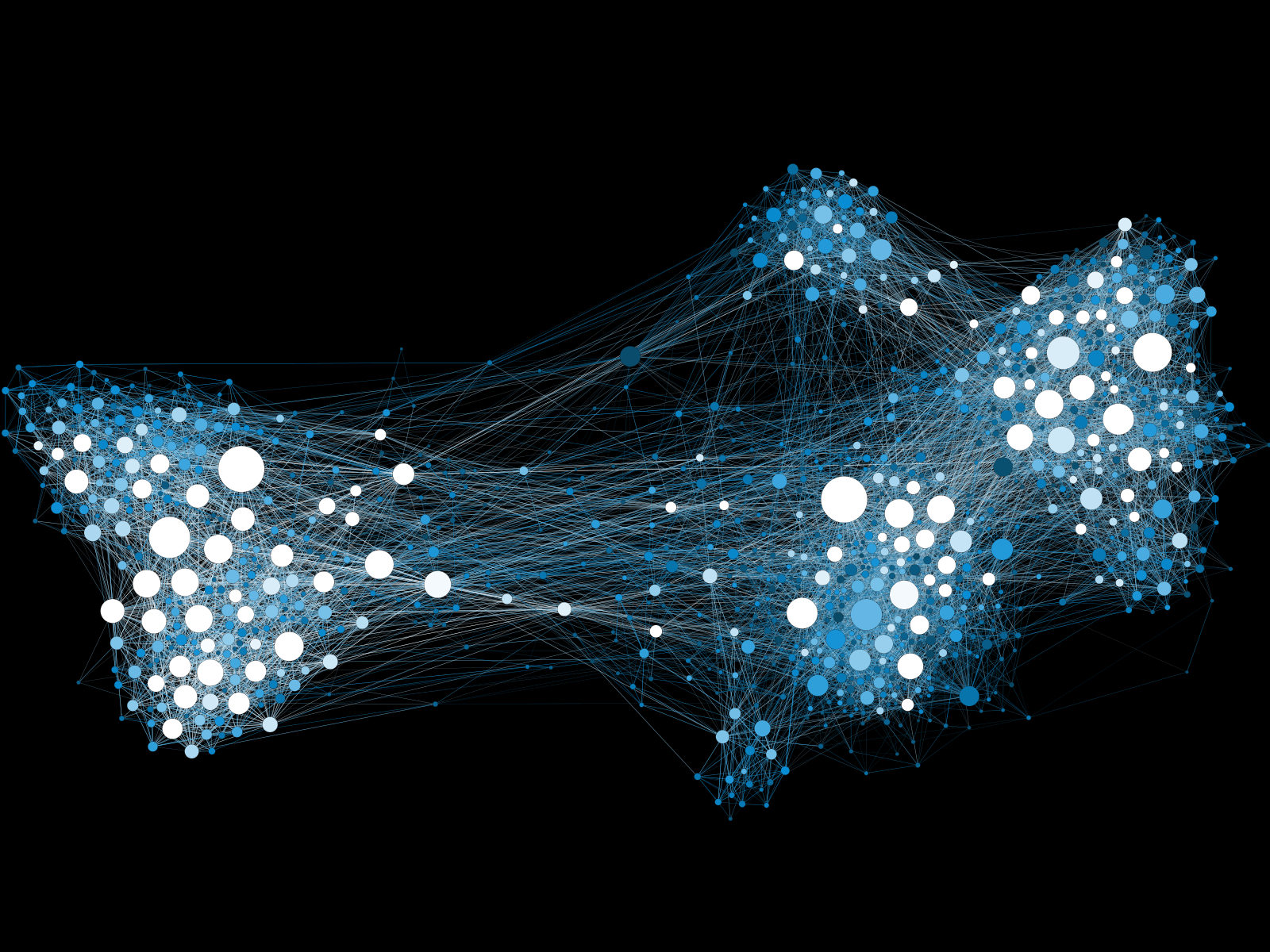
A generative AI model follows these maps to create something.
When you ask ChatGPT a question, it finds the closest matches to your words in its maps, then looks nearby. From that, it predicts what word or phrase is likely to come next. Then it repeats the process over and over to find good choices for the next word or phrase, and every one after that. To build an image, Midjourney predicts the most likely color of the next pixel or group of pixels, again and again.
These predictions involve some degree of chance. So the model follows a slightly different path through its map every time. That means the same prompt will give slightly different results each time.
Do you have a science question? We can help!
Submit your question here, and we might answer it an upcoming issue of Science News Explores
Fine-tuning for safety and honesty
A generative AI model doesn’t really understand the words, images or sounds it is creating. Without some additional training, it could easily spit out offensive, incorrect or harmful content. AI developers work to prevent this. That process is called fine-tuning.
The most popular way to fine-tune a generative AI model is to use people’s feedback. In this process, people look at AI’s responses to a prompt and choose the ones that they prefer. This process essentially makes certain paths through the model’s map much easier to follow. At the same time, other paths become more treacherous.
Once a model is fully trained, developers may also add rules or filters that check people’s prompts. These can prevent a model from answering certain unsafe or problematic prompts.
Putting too many restrictions on a bot can reduce its usefulness and creativity, though. So developers try to find a balance. People who use generative AI should be aware of its drawbacks as well as its opportunities.